Predictive Analytics in Performance Marketing: Why, What For, and How
Today, Olga Vyazovskaya, an executive director at the DV Group agency, shared her thoughts on predictive analytics in performance marketing. We’ll discuss key points in predictive analytics and examine Olga’s case study. You can find the original article by following the link.
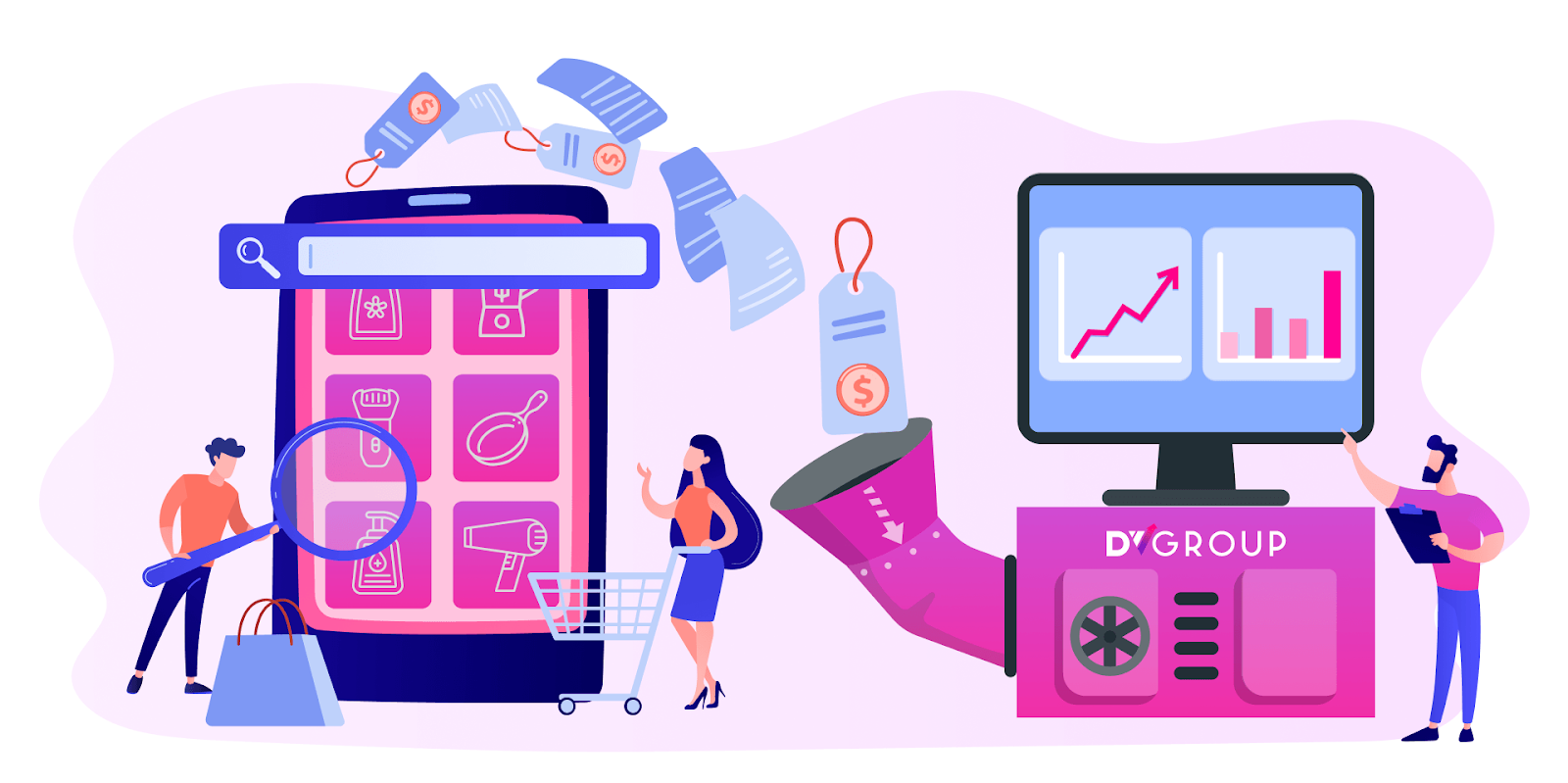
How wonderful it would be if we knew in advance that a pandemic was coming. Companies would calmly transfer employees to remote work and optimize their business for an online format. Yes, of course, making a forecast for this type of event is not easy. When it comes to business, forecasting today is the key to the success of the company, but how to make the forecast as accurate as possible? Predictive analytics comes to the rescue here.
So, predictive analytics is when we try to predict the future using scientific methods and disciplines: game theory, statistics, machine learning. The scope of the approach is wide: starting from logistics and banking, ending with marketing.
Imagine that we need to find out how likely the customer is to make a purchase. But how to do it when we don't know his tastes and needs. We don’t need this information if we use statistical and analytical methods that allow you to find patterns in historical data and predict the user’s further actions.
In this article, I’ll tell you how and why we use predictive analytics in the DV Group performance agency: how we calculate the probability of making a purchase of a certain category or brand in online stores, and how we form segments based on the data obtained for further traffic purchases.
Data Sources: Receipts and Cookies
The practical application of predictive analytics entails the optimal use of the marketing budget when conducting performance campaigns. Usually, marketers buy user interest-based traffic to expand the first stage of the sales funnel. However, if the information about user interests is not obtained based on the analysis of the consumer basket, it can lead to a negative ROI and a high CPO. That's why we use "advanced" segmentation to differentiate the bids in the advertising cabinet depending on the value of the user.
To illustrate our approach, let's break it into several parts:
Data sources and types of products they are suitable for.
We use receipt data and cookies from online stores as data sources.
Firstly, it is important to note that the receipt data can be used in mathematical models only for replenishable everyday goods, for example, beauty and personal care products, home cleaning, children's goods, food, and others. In case you’re dealing with long life cycle products, cookies showing the user’s actions before purchasing on the website are the only suitable option.
Secondly, in order to obtain a correct forecast, the limitation and volume of the processed data are important. When using receipts— you obtain a set of data for at least one year, provided that the number of transactions is at least three thousand pieces. When using cookies — at least three months and the number of transactions starting with ten thousand events.
Thirdly, the format of the received data. For example, for receipts, we use only raw source data without categorization. Because of this, we obtain a high degree of error during segment formation. In order to minimize the error, we categorize each SKU based on the faceted classification of the retailer, which practically eliminates attribution errors of goods to a certain category.
Process Modeling
The modeling process itself is divided into several parts:

To build the basic model, we use two approaches:
1. Solving a regression problem using a neural network based on LSTM.
Recurrent neural networks (RNNs) are networks that take into account previous information. LSTM (long short-term memory) is a type of recurrent neural network capable of learning long-term dependence. Their specialization is memorizing information for long periods of time.
All recurrent neural networks have the form of a chain of repeating neural network modules.
In standard RNN, this repeating module has a simple structure, and the LSTM module consists of four interacting layers. Due to this, there is no disconnection between the information, which allows us to determine the probability of a user making a purchase within 21 days.
The reason why it’s needed: suppose you need to buy a shampoo for your hair, it's more convenient for you to do it through an online store. You go to an online store and start searching by typing "shampoo" in the search bar, then falling into the category "hair care", sorting products by rating and checking the product cards, reading reviews, product characteristics, and, as a result, making a purchase. You spent, let’s say, two minutes choosing a shampoo. Most of the users who bought shampoo had spent the same amount of time. Thus, using mathematical models, you can predict the probability of a user making a purchase and even re-purchasing a product.
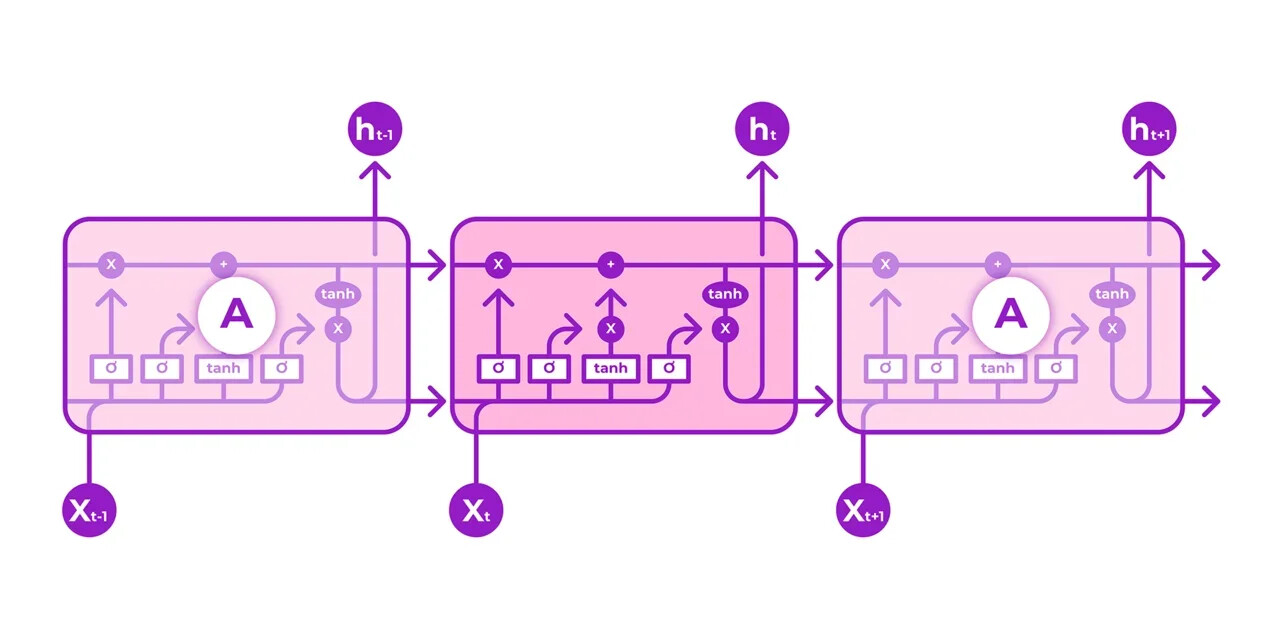
2. Latent analysis using matrix factorization.
Latent semantic analysis is a natural language information processing method that analyzes the relationship between a set of documents and the terms that occur in them.
With the help of analysis, it is possible to identify the characteristic factors inherent to all documents and terms. In other words, this approach allows you to fill in the "gaps" in the cells for the new object based on historical values. After applying these models we can analyze the probability of an event.
3. Scripting languages.
All our models are written in Python, data processing takes place inside the contour of our own DMP platform, with the further shipment of segments to advertising cabinets via the API. In the near future, we plan to switch to the CDP platform, which will allow us to combine all processes in one place.
How We Used Predictive Analytics When Launching an Advertising Campaign for Diapers Company
Let's take a specific example to see how predictive analytics works. The project is under NDA, so we don't use the brand name.
Our task was to increase sales of diapers on the major ecommerce marketplaces by attracting a new audience of young mothers with children under 2 years old. Special attention had to be paid to the audience of pregnant women, starting from the 7th month of pregnancy, and mothers with children up to 6 months, since it's a period when consumer preference is formed for the remaining period of consumption. To form segments, we split the source data into several parts:
Segment 1: Current buyers of diapers and panties in size NB, S, M.
Segment 2: Current size L buyers.
Segment 3: Current XL buyers.
Segment 4: Current buyers of maternity products (for all SKUs that fall into the faceted unloading of this category in online retailers).
Based on the resulting data samples and hypothesis testing, the following strategies were used:
- The use of current buyers of diapers and maternity products with no additional filters or segmentation.
- Building a lookalike audience using/based on the segments described above.
- Building a lookalike audience of users from these segments who are most likely to purchase the product within 21 days.
At the same time, it is important to note that the selected strategies were used within each of the segments. This was necessary for us to further differentiate the rates in advertising cabinets because the CPO for a new client from segments 1 and 4 can be three times higher than in segments 2 and 3.
We've placed ads on Facebook, Google, and Instagram. The average ROI when using a strategy with predictive modeling was 7.5, despite the fact that the segments of current buyers without additional processing turned out with an indicator of ROI = 2.1.
Of course, when choosing a strategy, it is necessary to take into account the capacity of the obtained segments, which is reduced due to additional filters, so we recommend using a combined scheme of work using several hypotheses simultaneously to achieve optimal ROI.
We use predictive modeling to divide users into precise segments As a result, our customers get the opportunity to increase sales on marketplaces and through their own online stores.
You can collect a segment of not just potential buyers of a store, category, or brand, but also users who are most likely to buy a specific product, and also find similar ones on the Internet, thus expanding the sales funnel at the entry.
The link to the original post: https://vc.ru/marketing/304609-prediktivnaya-analitika-v-performance-marketinge-pochemu-zachem-i-kak
500+ data sources under one roof to drive business growth. 👇