Enterprises often struggle to quickly deploy marketing intelligence due to inconsistent data formats and a fragmented advertising landscape. These inconsistencies make it challenging to gain timely and accurate insights, delaying decision-making and reducing the overall effectiveness of marketing strategies.
With data standardization, companies can streamline integration, enhance data accuracy, and speed up the deployment of actionable marketing intelligence.
This article examines what it means to standardize data and outlines effective strategies for implementing data standardization to fully leverage its advantages.
What Is Data Standardization?
By standardizing data, marketing teams can improve data quality, enhance cross-channel analytics, and support more informed decision-making.
Understanding Data Standardization and Related Concepts
Data standardization is often mixed up with several related but distinct terms, especially in the fields of data management and analytics. Understanding the differences between these terms is crucial for proper data handling and implementation of processes.
Here are the key terms that are commonly confused with data standardization.
Data Normalization
Data normalization refers to the process of organizing data in a database to reduce redundancy and improve data integrity. It involves structuring a database according to a set of normal forms to minimize duplication of information.
Data Cleansing (or Cleaning)
Data cleansing involves detecting and correcting (or removing) corrupt or inaccurate records from a dataset. This process includes identifying incomplete, incorrect, inaccurate, or irrelevant parts of the data and then replacing, modifying, or deleting this dirty data.
Data Enrichment
Data enrichment refers to the process of enhancing, refining, or improving raw data by merging third-party data from additional external sources. This process adds value to the original data set before it is used or analyzed.
Data Transformation
Data transformation is the process of converting data from one format or structure into another. This is a broad term that can include standardization but also encompasses other modifications like changing data types, aggregating information, and more complex computations.
Data Integration
Data integration involves combining data from different sources to provide a unified view. This process often involves various operations such as data transformation, cleansing, and enrichment to consolidate disparate data.
Why Standardize Data?
Inconsistent data formats cause inefficiencies, as teams waste time reconciling and cleaning data instead of focusing on strategic tasks. This often leads to missed opportunities due to inaccurate insights, making it difficult to properly analyze customer behavior and campaign performance. Additionally, unreliable data can damage customer relationships by negatively impacting personalization and service quality.
If this alone isn't enough of a reason to standardize data, here are more benefits that come with it.
Improved Data Quality
Standardizing data ensures that all information follows consistent formats and definitions, reducing errors and inconsistencies. For marketing specialists, this means more reliable data that can be trusted for analysis and decision-making, leading to more accurate insights and effective strategies.
Enhanced Cross-Channel Analytics
With standardized data, marketing teams can seamlessly integrate information from various platforms. This integration provides a comprehensive view of customer interactions and campaign performance across all channels, enabling more effective cross-channel analytics.
Streamlined Reporting
Data standardization simplifies the reporting process by ensuring that all data is comparable and aligned. This makes it easier to generate consistent and accurate reports.
Operational Efficiency
Standardized data reduces the time and effort required for data cleaning and preparation. This operational efficiency allows marketing specialists to focus more on strategic activities rather than spending valuable time on data reconciliation tasks, enhancing productivity and effectiveness.
Regulatory Compliance
Maintaining standardized data helps ensure compliance with data protection regulations such as GDPR and CCPA. By adhering to consistent data formats and definitions, marketing teams can better manage data privacy and security, reducing the risk of legal issues and fines.
Now that you know all the benefits of data standardization, let's move on to how to do it.
Data Standardization Implementation Strategies
Implementing data standardization effectively across an organization requires a well-thought-out strategy that addresses both the technical and human aspects of data management. Here are key steps to consider.
1. Establish Clear Standards
Begin by developing a comprehensive set of data standards that define formats, units, and data integrity rules.
For instance, data standardization involves setting uniform formats for customer contact information, such as ensuring all phone numbers are in the international format (e.g., +1-555-555-5555). Without standardization, the same phone number could be recorded in multiple inconsistent ways, such as 555-555-5555, (555) 555-5555, or +1 (555) 555-5555, which can cause issues when integrating data from various sources.
Collaboration is key, so involve stakeholders from various departments to ensure that these standards meet diverse functional needs and are adaptable across different platforms and technologies.
2. Leverage Automation Tools
Utilize automation tools to enforce these data standards consistently across the organization. Implementing marketing data pipeline and data management software will help automate the application of standardization rules during data entry and processing, minimizing manual errors and enhancing efficiency.
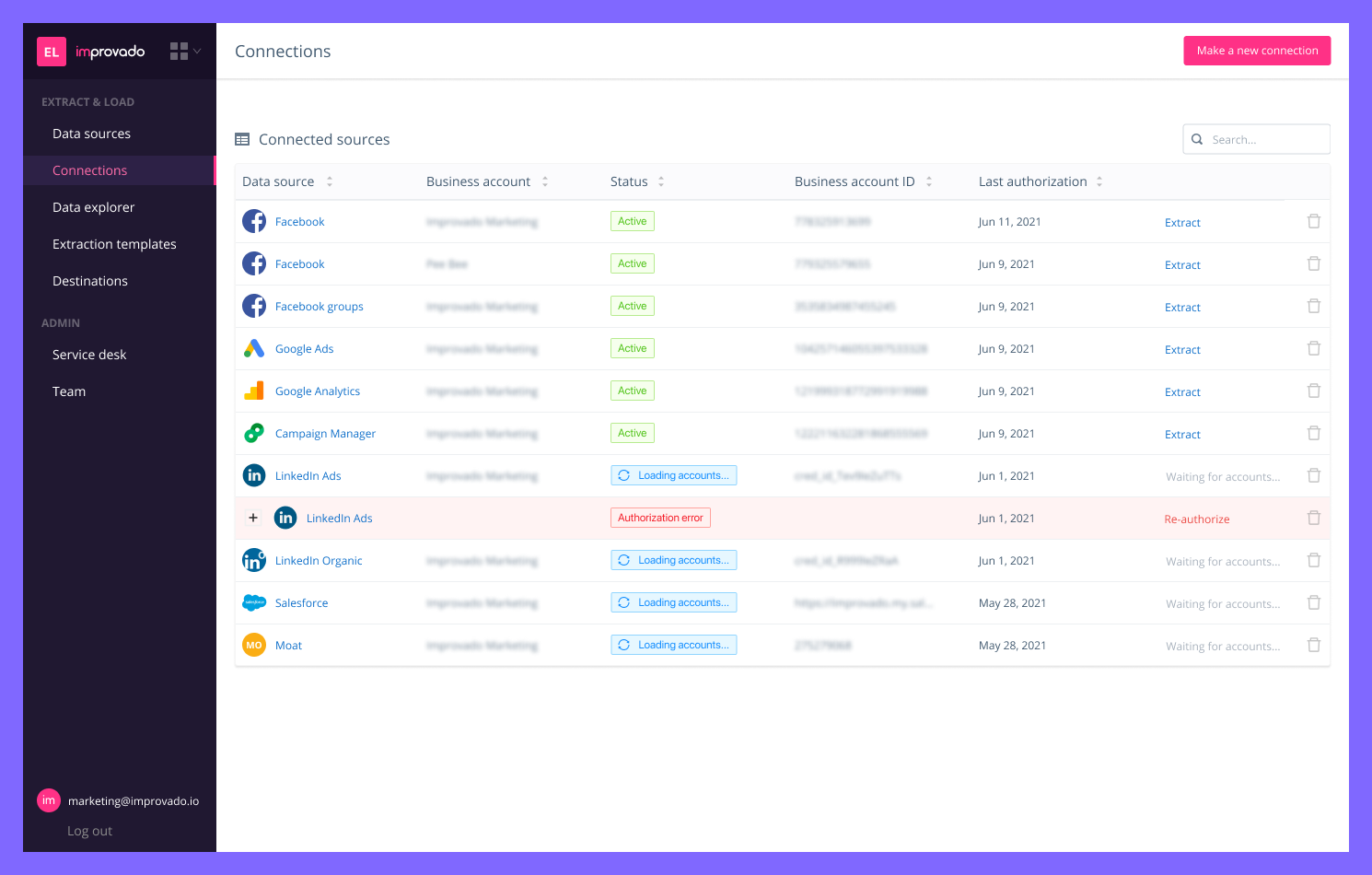
Improvado provides a solid data foundation for all-round marketing analytics and operations. The platform aggregates data from 500+ marketing and sales platforms, CRMs, and offline sources, automatically prepares it for analysis, and securely loads it to a data warehouse or a BI tool of your choice. The process is fully automated and doesn't require technical experience or knowledge of code.
3. Ongoing Training and Support
Data standardization is as much about people as it is about technology. Provide ongoing training for team members to understand the importance of standardization and ensure they are equipped to apply it effectively. Establish support channels to address any standardization issues, helping to maintain high standards and encourage adherence to established protocols.
4. Regular Quality Assurance
Conduct periodic audits and quality checks to verify that data standardization protocols are being followed and are delivering the expected benefits. Use the insights gained from these reviews to refine standards and processes continually.
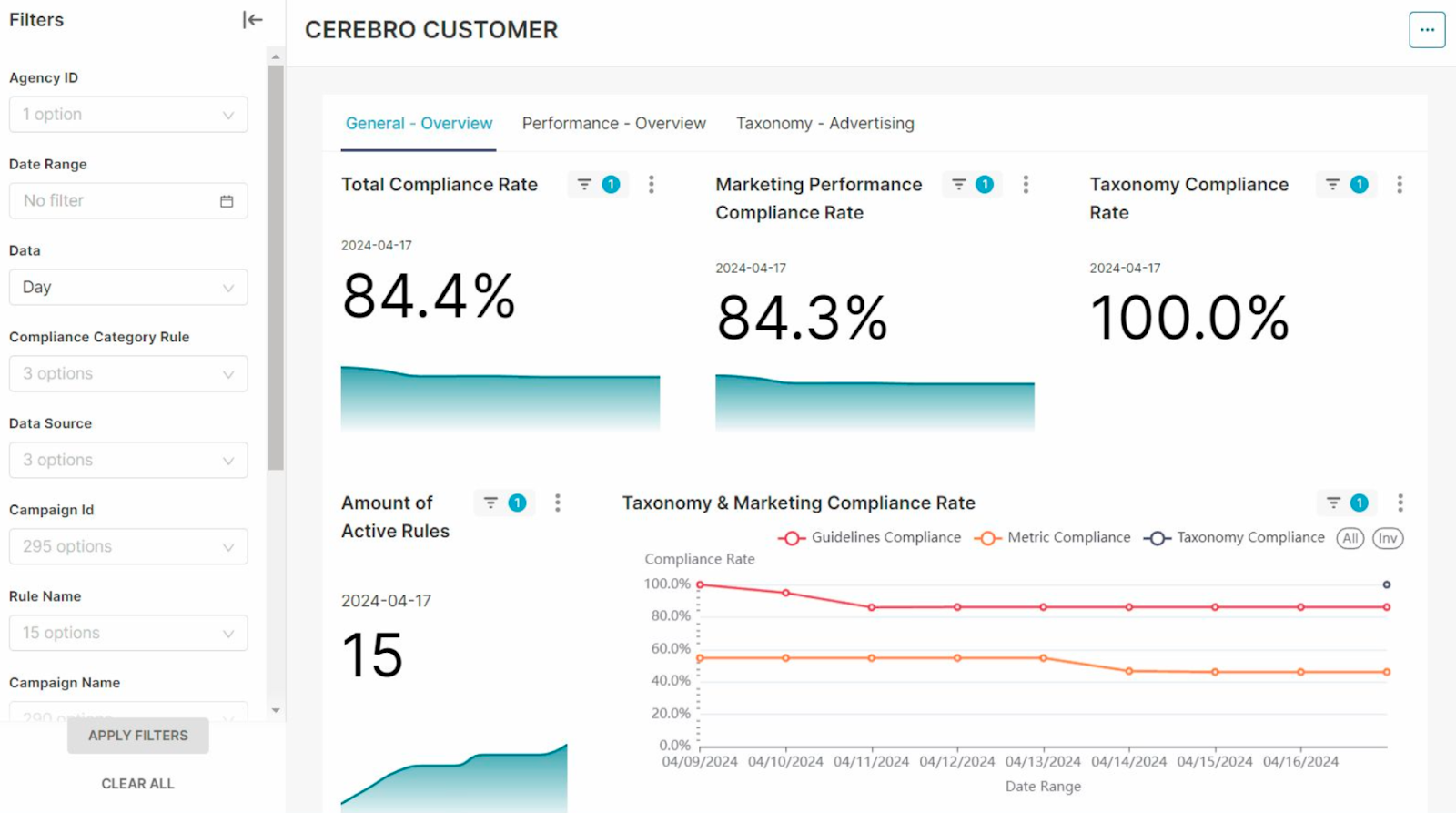
Additionally, streamline the process by integrating automated data quality management software solutions like Marketing Data Governance. Marketing Data GovernanceMarketing Data Governance is an AI-powered campaign management and data governance service that automatically validates the consistency of your data and alerts you of any anomalies and data discrepancies.
5. Scalable Infrastructure
Build a flexible and scalable infrastructure that can support adjustments and expansions to data standards as business needs evolve. This infrastructure should allow for easy updates and modifications without requiring extensive overhauls, thus supporting the dynamic nature of marketing data.
Data Standardization Challenges and Solutions
Implementing data standardization across a large enterprise involves overcoming significant challenges. Here’s how organizations can address these obstacles effectively.
Resistance to Change
Employees may resist adopting new data standards, especially if the benefits aren’t immediately clear or if it disrupts their usual workflows.
Solution
Engage and educate:
- Involve employees in the standardization process from the beginning. This includes gathering their input on pain points and involving them in the planning stage.
- Implement comprehensive training programs that explain the benefits of data standardization, not just for the company but for their individual roles. Highlight how it will make their work easier and more impactful.
Data Silos
Different departments may have their own data management systems and protocols, leading to fragmented data practices that are hard to unify.
Solution
Incremental integration:
- Create teams that include representatives from all critical departments. This helps ensure the standardization efforts consider all aspects of the company’s operations.
- Implement the standardization in phases across departments. Start with areas that are less complex or more receptive to change to build momentum and showcase early successes.
Maintaining Data Quality
Transitioning to a standardized format can initially lead to discrepancies in data quality, especially if the existing data is inconsistent.
Solution
Robust quality controls:
- Regular audits of the data should be conducted during and after the transition to identify and rectify any inconsistencies or errors.
- Employ continuous monitoring tools that automatically detect deviations from data quality standards, allowing for immediate corrections.
Scalability Concerns
As the business grows, the data standardization framework must scale efficiently without necessitating major overhauls.
Solution
Scalable Architecture:
- Utilize modular software design principles so that new features or updates can be added without impacting the existing system significantly.
- Leverage cloud technologies that offer scalability and flexibility to accommodate growing data needs and computational demands.
Technical Limitations
Older IT systems might not support the integration of new standardization protocols, creating a technological gap.
Solution
Advanced integration techniques:
- Implement middleware that can interface between old and new systems, translating data formats on the fly.
- Plan for gradual upgrades or replacements of outdated systems that align with the long-term IT strategy of the organization.
Optimizing Marketing Campaigns through Data Standardization
Improvado streamlines the process of data standardization by automating the entire data preparation cycle, from data extraction to normalization and transformation. The platform ensures that data from various channels is consistent and ready for analysis. This is crucial for organizations that depend on accurate and uniform data to inform their marketing strategies.
Marketing Data Governance by Improvado enhances these capabilities by focusing on campaign data management and data governance. Marketing Data Governance automates compliance and operational checks for marketing data against established standards, ensuring that everything from naming conventions to targeting parameters is consistent across all assets.
FAQs
What is data standardization?
What are some key benefits of data standardization?
How to standardize data?
What is the difference between data normalization vs standardization?
500+ data sources under one roof to drive business growth. 👇
Improvado provides access to accurate and timely insights