Data Quality Management: Tools, Implementation Strategies, and Best Practices
The reality is that if you want to improve performance, you not only need to make the right decisions but also base these decisions on accurate and up-to-date data. Therefore marketing teams often find themselves bogged down by tasks like data maintenance and validation instead of focusing on making decisions and optimizing campaigns.
Data quality management offers a framework that helps establish efficient processes for data maintenance, validation, and integration. By implementing these practices, marketing teams can ensure their data is accurate, consistent, and reliable and focus on tasks that matter the most.
What Is Data Quality Management?
An effective data quality management strategy enables marketing teams to trust their data, allowing them to focus on making strategic decisions without being sidetracked by data inconsistencies or inaccuracies.
It also helps in maintaining up-to-date and actionable data, which is essential for accurate customer segmentation, targeting, and performance measurement.
Data Quality Management vs. Data Governance
While often used interchangeably, data quality management and data governance serve distinct but complementary functions within an organization. Differentiating the two is crucial for marketing teams to ensure they implement the right strategies for managing their data effectively.
Data quality management focuses on the tactical aspects of managing data, including processes like data cleansing, integration, validation, and monitoring. DQM ensures that the data used for marketing campaigns, customer segmentation, and performance analysis is free from errors and discrepancies.
Data governance, on the other hand, is more strategic and involves the policies, standards, and procedures that govern how data is managed and used across the organization. It includes establishing data ownership, defining data policies, ensuring compliance with regulations, and setting the framework for data stewardship. Data governance ensures that data management practices align with the organization's goals and regulatory requirements.
Aspect | Data quality management | Data governance |
---|---|---|
Focus | Tactical data accuracy and reliability | Strategic data policies and compliance |
Key Processes/td> | Data cleansing, integration, validation, monitoring | Policy setting, data ownership, compliance, data stewardship |
Objective | Ensure data used in operations is error-free and consistent | Ensure data management aligns with organizational goals and regulations |
Responsibility | Data analysts, data quality teams | Data governance board, data stewards |
Outcome/td> | High-quality data for immediate use in marketing strategies | Long-term data integrity and regulatory compliance |
While data quality management and data governance have distinct roles, they are deeply interconnected.
Effective data governance creates the environment and conditions under which data quality management can succeed. Governance policies define what constitutes quality data within the organization, and data quality management ensures those standards are met through practical application.
The Importance of Data Quality Management for Enterprises
Poor data quality leads to inefficiencies, as teams spend excessive time correcting errors instead of focusing on strategic initiatives. It also results in missed opportunities due to faulty insights and can damage customer relationships when inaccurate information affects personalization and service quality. Inaccurate data can further lead to compliance risks and potential legal penalties, adding to the financial burden.
Additionally, stats show that 40% of business objectives fail due to inaccurate data.
These figures underscore the importance of robust data quality management to prevent financial losses, ensure accurate decision-making, and achieve business objectives.
Implementing an Effective Data Quality Management Program
Implementing an effective data quality management program is a complex and ongoing process that demands collaboration across multiple teams within an organization. A successful DQM program involves continuous monitoring, validation, and improvement of data quality to ensure its accuracy, consistency, and reliability. To achieve this, it is essential to involve data analysts, marketers, IT professionals, and key stakeholders.
1. Define Data Quality Objectives
Begin by clearly defining what data quality means for your organization. Set specific, measurable objectives that align with your overall business goals, such as improving customer data accuracy or reducing the number of incomplete records in your marketing campaigns. These objectives will guide the development of your data management strategies and serve as benchmarks for success.
To increase team engagement, elaborate on the objective by identifying key areas where data quality impacts your marketing efforts. For example, improving customer data accuracy can lead to better targeting and personalization in campaigns, resulting in higher engagement and conversion rates.
Additionally, establish clear metrics and KPIs to track progress towards these objectives. This might include measuring the percentage of accurate customer profiles, the rate of duplicate record elimination, and the timeliness of data updates.
2. Standardize Data Handling Procedures
Establish standardized procedures for how data is collected, processed, stored, and disposed of. These standards ensure consistency across the organization and reduce the likelihood of data errors. Clear guidelines also facilitate training and compliance, particularly important in environments with high staff turnover or multiple departments handling customer data.
3. Invest in Data Quality Management Tools
Leverage advanced data management tools that automate key processes such as data cleansing, validation, and enrichment. These tools can significantly enhance the efficiency and accuracy of your data quality initiatives by systematically addressing common data issues before they impact marketing results.
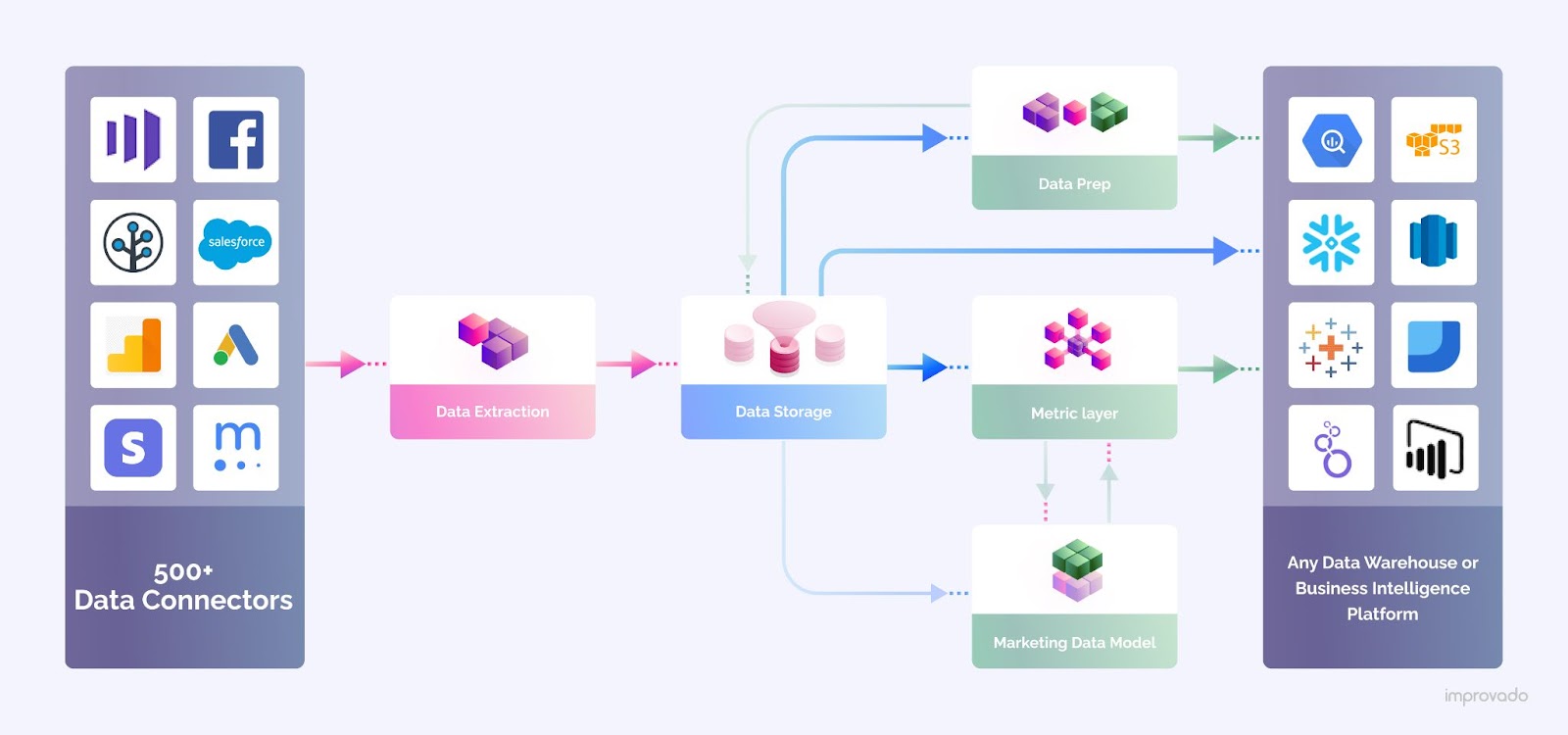
Improvado provides a solid data foundation for all-round marketing analytics and operations. The platform aggregates data from 500+ marketing and sales platforms, CRMs, and offline sources, automatically prepares it for analysis, and securely loads it to a data warehouse or a BI tool of your choice. The process is fully automated and doesn't require technical experience or knowledge of code.
4. Continuous Data Quality Monitoring
Implement continuous monitoring systems to track data quality over time. These systems can quickly identify and alert teams to issues as they arise, allowing for prompt resolution.
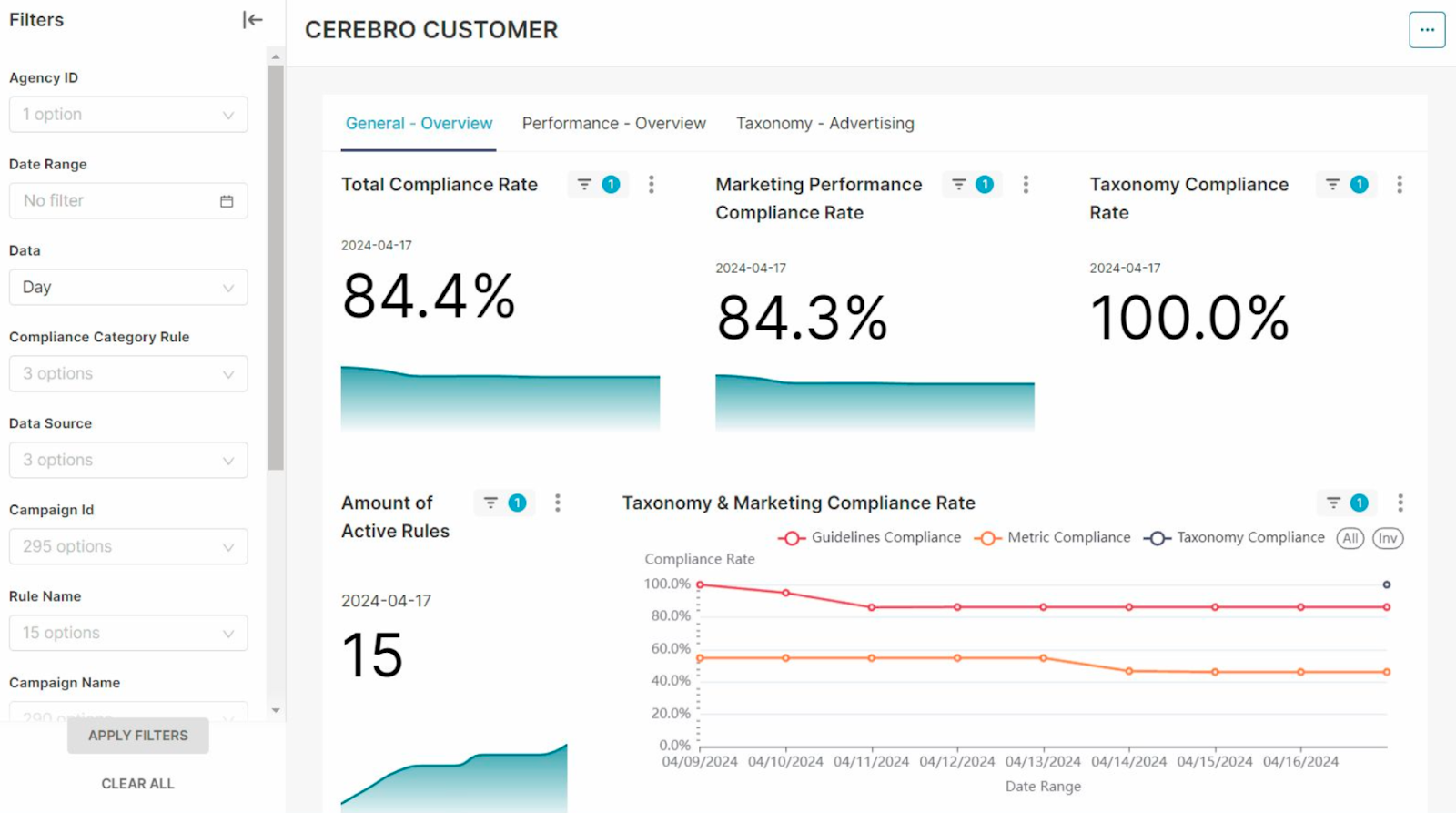
Streamline the process by integrating automated data quality management software solutions like Cerebro. Cerebro is an AI-powered campaign management and data governance service that automatically validates the consistency of your data and alerts you of any anomalies and data discrepancies.
5. Foster a Data-Quality Culture
Promote a culture where data quality is valued across the organization. Encourage teams by demonstrating how high-quality data can lead to more successful marketing campaigns and better business decisions.
Here are some practical steps and examples for fostering a data-quality culture:
- Leadership buy-in and advocacy: Senior leaders should actively support and advocate for data quality initiatives. This could involve incorporating data quality metrics into KPIs for leadership roles, highlighting the importance of accurate data in achieving business goals.
- Data stewardship programs: Establish data stewardship programs where specific individuals or teams are responsible for maintaining data quality within their domain. For instance, a data steward in the marketing department can oversee the accuracy of customer data and campaign metrics, ensuring that all data-related processes adhere to defined standards.
- Integrate data quality into daily operations: Ensure that data quality is integrated into everyday business processes. This might involve embedding data quality checks into workflow systems, using automated tools to flag errors, and requiring data validation steps before data entry or reporting. For instance, marketing automation platforms can include real-time data validation features that prompt users to correct errors immediately.
Elevating Data Quality Management with Cerebro
Improvado transforms data quality management by automating essential processes such as data collection, cleansing, validation, and compliance checks. This reduces the need for manual oversight and allows marketing teams to redirect their focus from data maintenance to strategic planning.
The tool's real-time monitoring capability is particularly beneficial, as it quickly identifies and addresses data inconsistencies. This immediate response prevents small errors from escalating into larger issues that could impact campaign performance.
The efficiency gained from using Improvado allows marketing professionals to spend more time analyzing data and less on managing it, supporting smarter, data-driven decision-making that can propel business growth.
FAQs
What is data quality management?
How does data quality management enhance business intelligence?
What are DQM tools?
500+ data sources under one roof to drive business growth. 👇
Improvado provides access to accurate and timely insights