A Step-by-Step Guide to Data Quality Audits
The 1-10-100 rule of data quality highlights the escalating costs associated with poor data management.
This rule emphasizes the importance of regularly conducting data quality audits and investing in data management from the outset to avoid exponential costs related to it.
What Are Data Quality Audits?
This process typically involves checking for duplicate records, verifying the correctness of data fields, and ensuring that data aligns with real-world scenarios. By conducting regular data quality audits, marketing teams can identify and rectify issues that could lead to ineffective targeting, inaccurate reporting, and suboptimal campaign outcomes. This ensures that all marketing efforts are based on reliable and high-quality data, ultimately enhancing the effectiveness of marketing strategies and maximizing ROI.
Planning a Data Quality Audit
Effective planning is the foundation of a successful data quality audit. This phase involves setting clear objectives, identifying the data to be audited, and defining the key metrics and standards.
1. Set Clear Objectives for the Audit
Determine what you aim to achieve, such as identifying inaccuracies, inconsistencies, and gaps in the data. Specify the scope of the audit, including the data sources, types of data, and specific datasets to be reviewed.
2. Identify Key Data Elements
Focus on the critical data elements that impact marketing activities. This could include customer contact information, transaction data, campaign performance metrics, and segmentation criteria. Prioritize data elements based on their importance to marketing operations and decision-making.
3. Establish Data Quality Criteria
Define the standards and criteria for data quality. Common criteria include accuracy, completeness, consistency, timeliness, and relevance. Establish benchmarks and acceptable thresholds for each criterion to measure the quality of your data.
4. Develop an Audit Plan
Create a detailed plan outlining the audit process, including timelines, responsibilities, and specific tasks. Include procedures for data collection, analysis, and reporting. Ensure the plan is flexible enough to accommodate any unexpected challenges or findings.
The Data Quality Audit Process
The data quality audit process typically involves three key steps: establishing data quality metrics, collecting and analyzing data, and identifying and documenting data quality issues.
1. Establish Data Quality Key Metrics and Standards
Defining the metrics that will be used to assess data quality is the first step. Common metrics include:
- Accuracy: The degree to which data correctly describes the real-world entity or event.
- Completeness: The extent to which all required data is present.
- Consistency: The degree to which data is the same across different data stores.
- Timeliness: The degree to which data is up-to-date and available when needed.
- Uniqueness: The degree to which data is free from duplicates.
2. Data Collection and Analysis
Gathering the data from the selected datasets and performing a detailed analysis is the next step. This involves profiling the data to understand its structure and quality. Tools and techniques such as pattern analysis, redundant data analysis, and null count assessments can help identify potential issues.
3. Identify and Document Data Quality Issues
Common data quality issues include:
- NULL values: Fields left blank due to errors or omissions. For example, missing customer email addresses in a marketing database can lead to ineffective email campaigns.
- Schema changes: Discrepancies caused by changes in data structure. For instance, a change in the format of a date field from "MM/DD/YYYY" to "YYYY-MM-DD" without updating the corresponding data pipeline can cause errors in data processing.
- Volume issues: Unexpected variations in data volume. An example is a sudden spike in website traffic data due to a bot attack, which can skew analytics reports.
- Distribution errors: Data that falls outside acceptable ranges. For example, an unusually high number of clicks from a single geographic location might indicate fraudulent activity.
- Inaccurate data: Incorrect data entries or values. An example is an extra zero added to a revenue figure, turning $10,000 into $100,000, which can lead to misinformed financial decisions.
- Duplicate data: Multiple instances of the same data. For instance, having multiple records for the same customer can result in double-counting and inaccurate customer insights.
- Relational issues: Problems with relationships between different data entities. For example, orphan records in a database where transactions exist without corresponding customer records can lead to incomplete analysis.
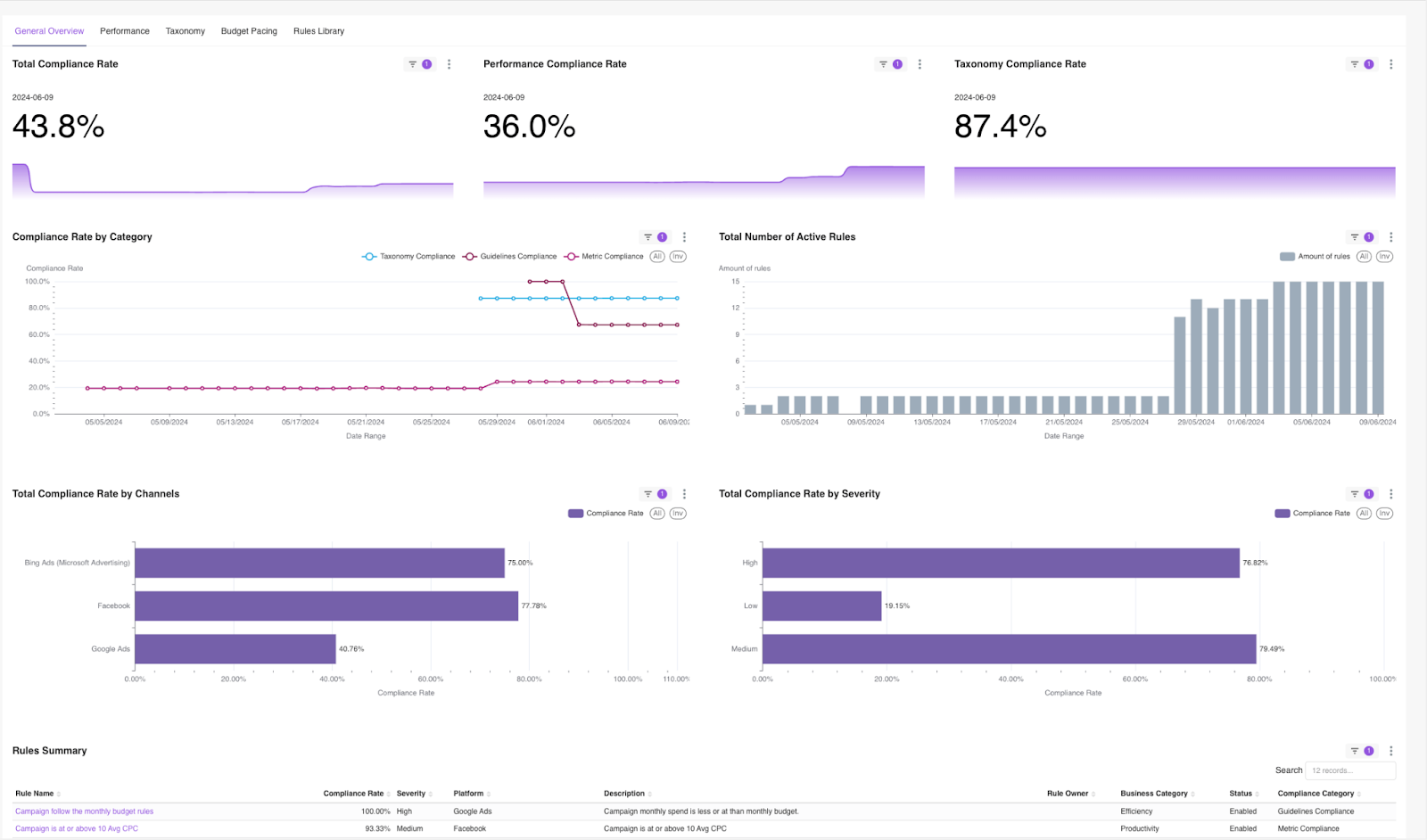
Post Data Quality Audit Actions
Once the data quality audit is complete, developing a remediation plan and implementing continuous monitoring is crucial to maintaining data quality.
1. Develop a Remediation Plan
Based on the audit findings, creating a plan to address the identified issues is essential. This plan should include specific actions for correcting data inaccuracies, eliminating duplicates, and updating incomplete data. Data transformation and validation capabilities in analytics platforms can automate many of these remediation steps, making the process more efficient and effective.
2. Implement Improvements and Monitor Continuously
Implementing the remediation actions and establishing a system for continuous data quality monitoring is the final step. Using data observability tools to automate monitoring processes and quickly detect and address any new data quality issues can be very effective.
Integrating Improvado for Enhanced Data Hygiene
Integrating Improvado into your data management workflows significantly improves the quality of your data by automating the aggregation, transformation, and validation of data from various marketing and sales platforms. This automation ensures that your data is consistently formatted and up-to-date, reducing the risk of errors that can arise from manual data handling. Improvado helps maintain a high level of data quality across all sources, making it easier to analyze and make accurate data-driven decisions.
FAQs
500+ data sources under one roof to drive business growth. 👇
Improvado provides access to accurate and timely insights