Operating in a data-rich and competitive environment, businesses face the dual challenge of managing an overwhelming amount of data while also seeking ways to use this data as leverage for growth and success.
This article explores data-driven decision-making as a critical strategy that allows brands to transform data from an overwhelming burden into a valuable asset. It highlights the importance of collecting, analyzing, and applying data systematically to inform strategic decisions, navigate market complexities, and secure a competitive edge.
What Is Data-Driven Decision Making?
The crux of data-driven decision-making lies in its capacity to eliminate guesswork, enabling marketing decision-makers and other business users to lean on verifiable data trends and metrics for making decisions.
Benefits of Data-Driven Decision-Making Approach
At its core, this approach enhances strategic decision-making by grounding actions in data analysis, leading to several key advantages:
- Improved accuracy: Data-driven decisions reduce reliance on intuition, minimizing the risk of bias and error. By basing strategies on factual insights, companies can target their efforts more effectively.
- Enhanced efficiency: Automating data collection and analysis speeds up the decision-making process, allowing businesses to respond to market changes swiftly and capitalize on emerging opportunities.
- Increased revenue: By identifying trends and customer behaviors through data, businesses can tailor their offerings and marketing strategies to meet demand more accurately, often resulting in increased sales and customer satisfaction.
- Cost reduction: Data-driven insights help identify areas of waste and inefficiency, allowing companies to streamline operations and reduce costs without compromising on quality or customer experience.
- Competitive advantage: Access to timely and accurate data insights can set a company apart from its competitors, enabling it to anticipate market shifts, adapt strategies accordingly, and meet customer needs more effectively.
Key Steps in the Data-Driven Decision-Making Process
While the data-driven decision-making approach might seem straightforward — simply rely on metrics to guide decisions — the reality is quite complex.
From the initial collection of raw data to the final transformation into actionable insights, this process involves multiple intricate steps. Each phase is critical, requiring careful execution to ensure the data accurately informs strategic choices.
This section breaks down these steps.
1. Identify objectives
The first critical step in the data-driven decision-making process is to define clear, quantifiable targets that the organization seeks to achieve.
For example, a marketing team might set an objective to increase website traffic by 20% within the next quarter. This specific goal then directly influences the selection of relevant data for analysis, such as historical website traffic patterns, sources of current web visitors, and the effectiveness of previous traffic-boosting initiatives.
Moreover, the defined objective guides the choice of analytical methods and tools. In our example, the team might employ web analytics tools to segment traffic by source and analyze user behavior on the site.
2. Data collection
Once objectives are set, the focus shifts to collecting relevant data. This involves identifying internal and external data sources that can provide insights related to the objectives.
For a marketing team aiming to increase website traffic (if we go with our example), this might involve collecting data on Google Analytics 4, Google Search Console, Semrush, Bing Webmaster, social media platforms, and others.
The integrity of the data collection process is critical. Ensuring the quality, reliability, and timeliness of data is non-negotiable, as these attributes significantly influence the outcome of the analysis. Verification of data sources is a must to guarantee that the information used is accurate and dependable.
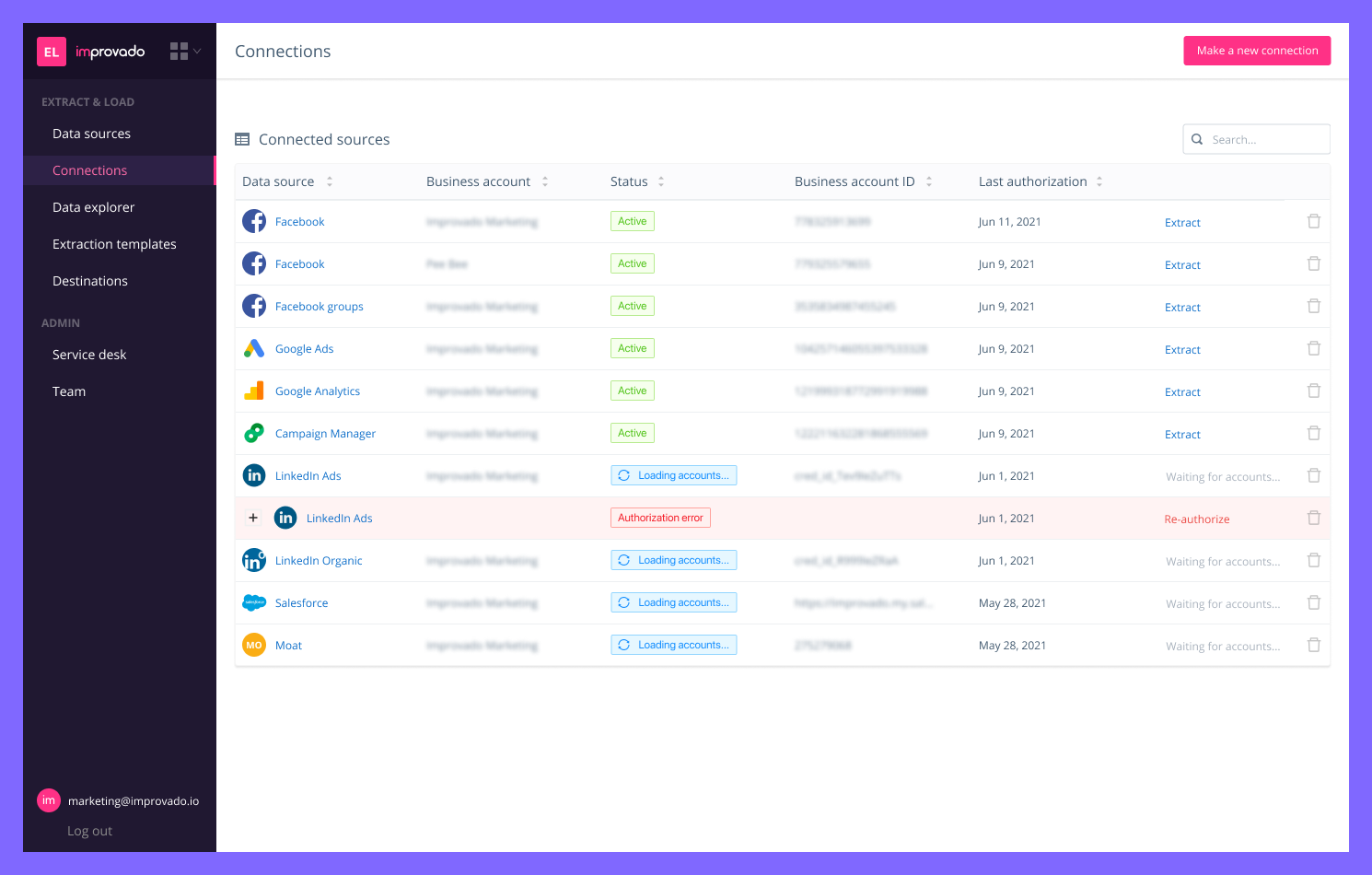
3. Data processing
After collection, data must be processed to ensure it's in a usable state for analysis. This includes cleaning data to remove errors, inconsistencies, and duplicates, as well as organizing it in a structured format. Effective data processing reduces noise in the dataset, making the analysis more focused and efficient.
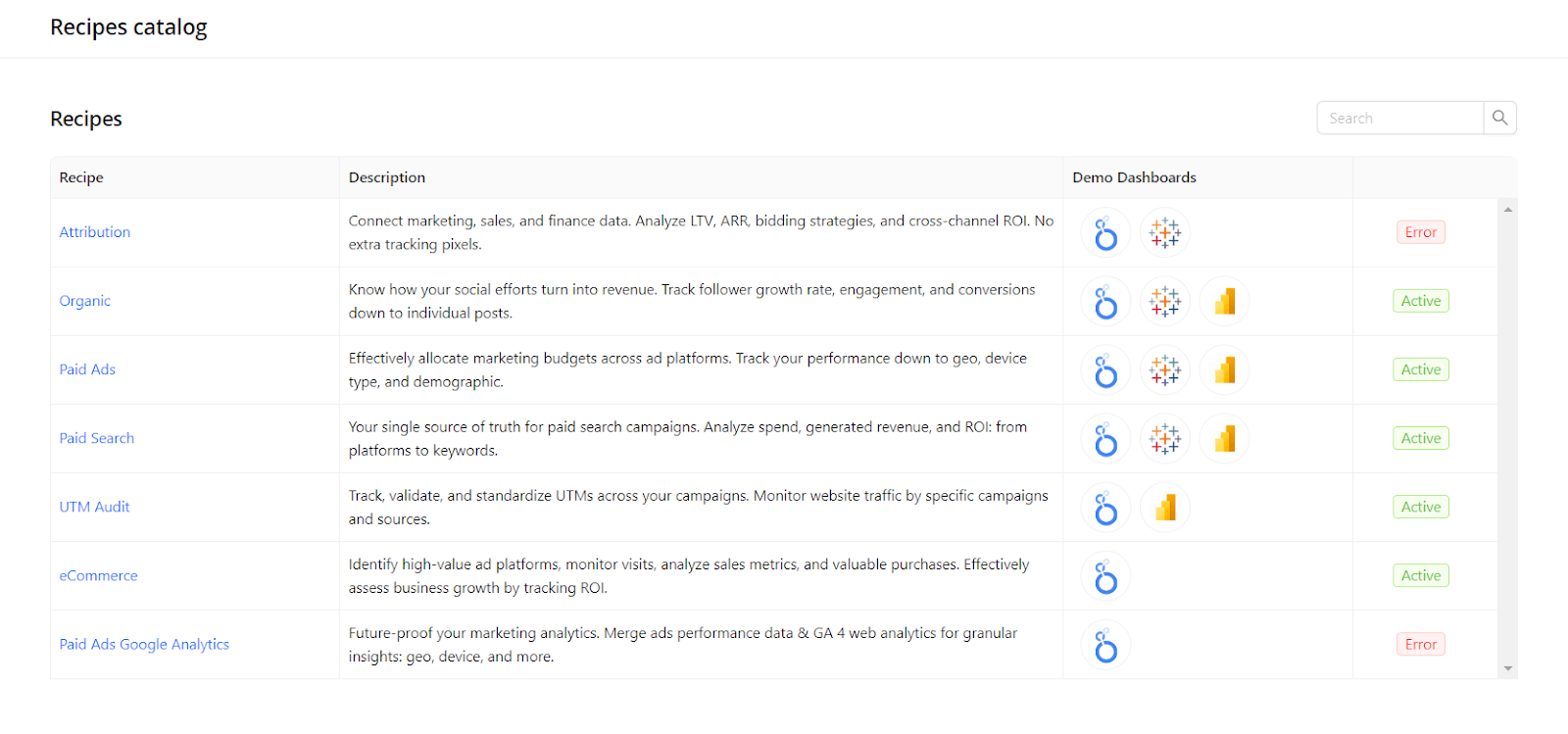
Improvado streamlines data processing by cleaning, normalizing, and mapping data without the need for manual intervention or custom scripts. The platform provides two options:
- Pre-built data pipelines from multiple marketing use cases spanning from data extraction to visualization for various use cases. For instance, if you select a paid ads analytics recipe, the platform will extract the needed data from the ad platforms, automatically map the platform's unique spend structures, and present a dashboard with data on daily campaign performance down to adset, ad level, creative, or placement level.
- Self-service data transformation engine that has a spreadsheet-like UI and supports over 300 features and functionalities to automate lengthy analytics timelines and facilitate data discovery.
4. Data analysis
With processed data in hand, the next step is to analyze it to extract meaningful insights. In practice, data analysis can be applied in various ways, from predictive analytics and revenue attribution to complex customer segmentation and AI-powered data exploration.
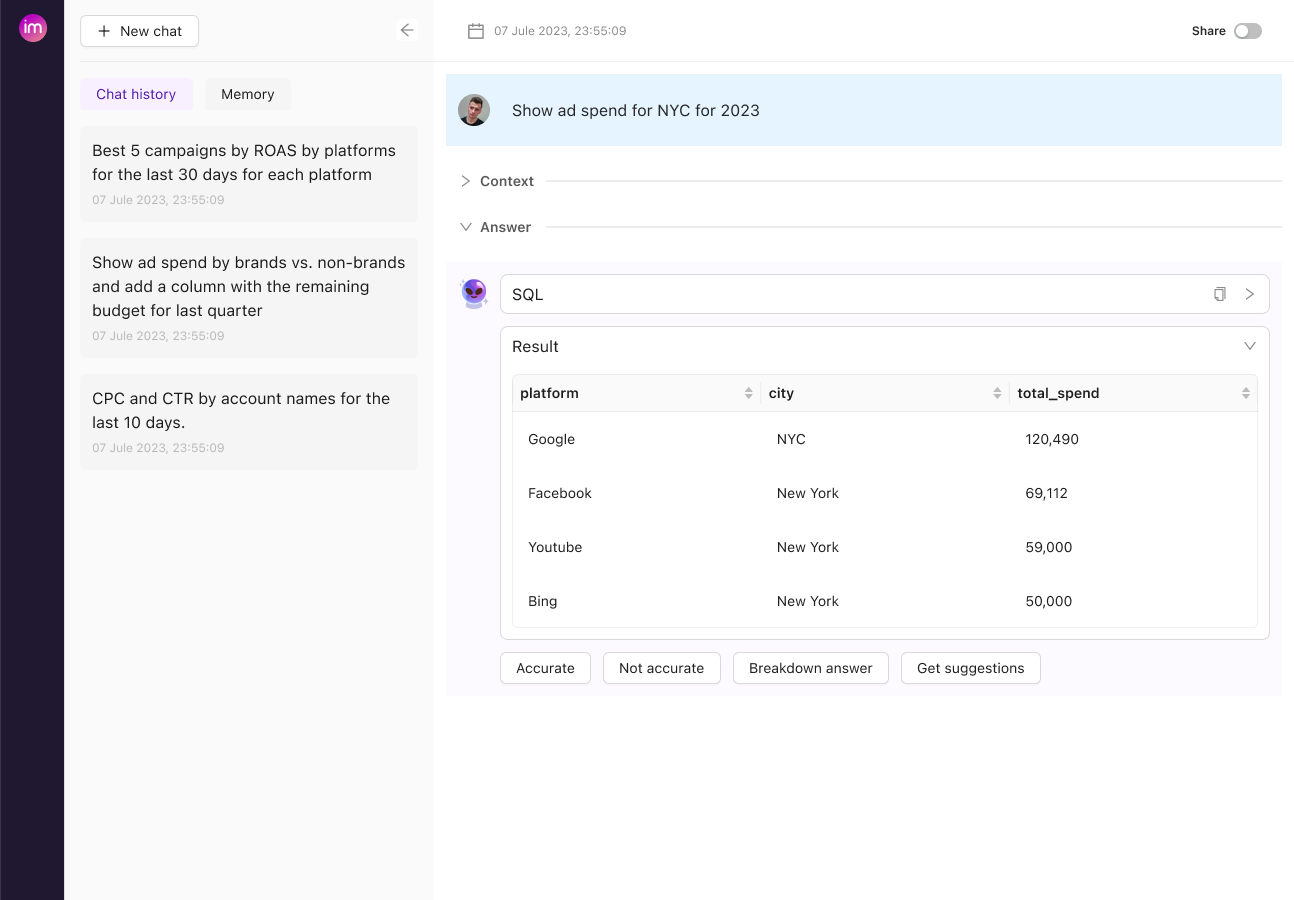
The complexity of analysis can vary, but the goal remains the same: to derive actionable insights that align with the predefined objectives.
5. Interpretation
Interpreting the results of the data analysis is where data turns into decision-making power. This step requires translating complex data findings into understandable insights that have clear implications for business strategy. It's crucial to consider the business context to ensure that the interpretations are relevant and actionable.
6. Decision-making
Armed with interpreted data, decision-makers can now make informed choices. This involves evaluating the implications of various options and selecting the strategies that best meet the organizational goals. Decisions should be supported by data but also consider qualitative factors such as company values and market conditions.
Let's get back to our website traffic example. If the data reveals that certain types of content drive more traffic, the decision might involve allocating more resources to content development in those areas. If an analysis suggests heavy investment in paid advertising, but the company prioritizes organic growth, the strategy might be adjusted to balance both aspects. Similarly, if market conditions indicate a surge in interest in certain topics or products, efforts might be directed toward capitalizing on these trends.
7. Implementation
Following the decision, the chosen strategy or action is implemented. This stage requires detailed planning and coordination to ensure that the decision is executed effectively.
8. Evaluation
The final step is to evaluate the outcomes of the decision against the initial objectives. This involves measuring performance indicators, analyzing the impact of the decision, and gathering feedback.
Practical Examples of Data-Driven Decision-Making in Action
To see how data-based decision-making can really change the game, let's check out five real-world examples where this approach makes a big difference.
1. Optimizing marketing campaigns
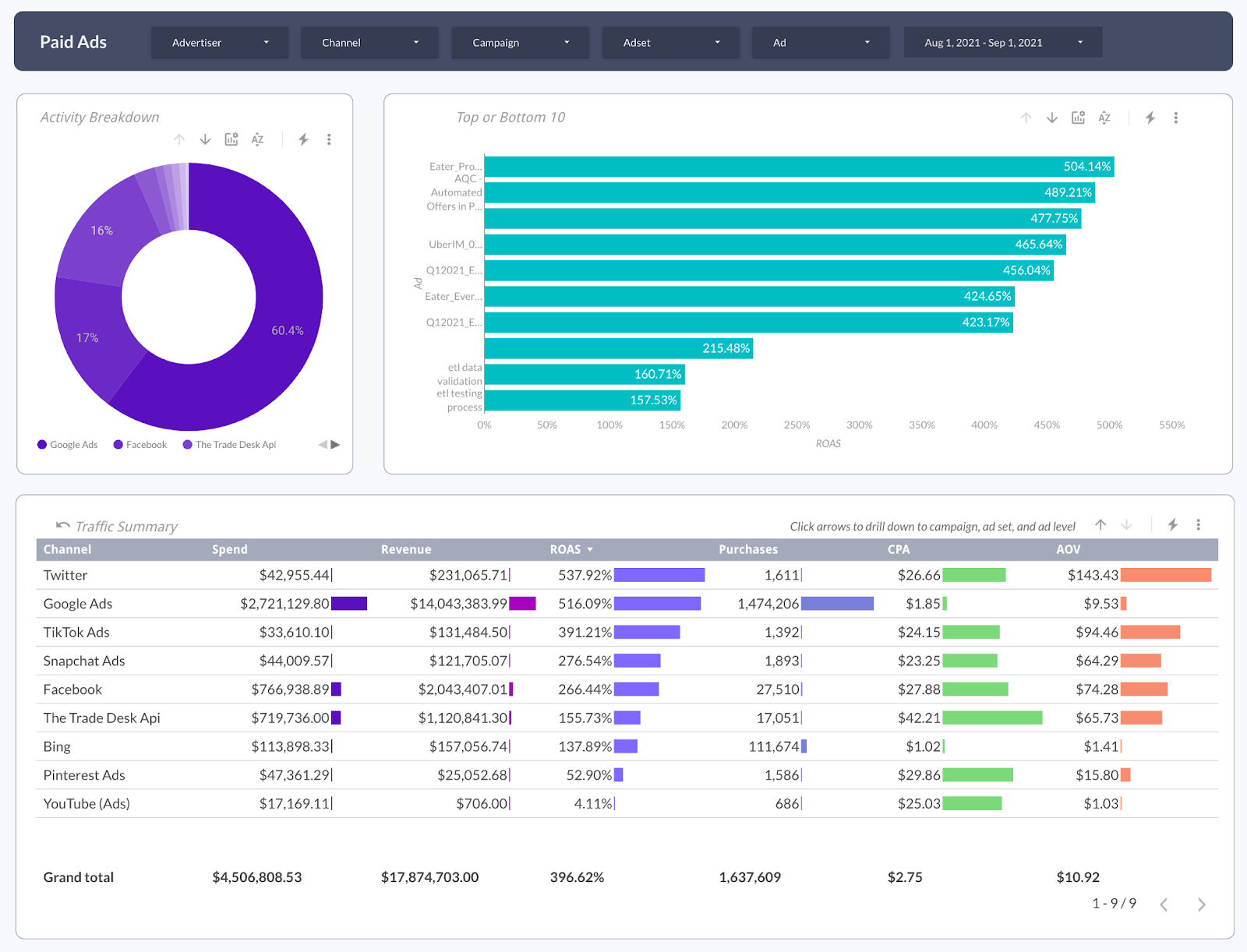
A clear illustration of data-driven decision-making comes from the optimization of advertising campaigns. By delving into data on ad performance across different platforms, times, and audience segments, marketers can identify which combinations yield the best results in terms of engagement, click-through rates (CTR), and return on investments.
2. Personalizing customer experiences
Through the strategic use of customer data—ranging from demographic information and purchase history to online behavior and engagement patterns—companies can tailor interactions and offerings to meet individual customer needs and preferences.
For instance, by analyzing purchase history data, a company can identify patterns in customer preferences for certain products or services. This insight allows for the customization of marketing messages and offers to match these preferences, significantly increasing the relevance and effectiveness of communications.
Moreover, website and app usage data provide a wealth of information on customer behavior, including the most visited pages, time spent on specific content, and interaction with various elements. By leveraging this data, businesses can optimize the user experience on their digital platforms, presenting personalized product recommendations, content, and offers that resonate with individual users’ interests and behaviors.
3. Predictive sales forecasting
By analyzing patterns in past sales data, market conditions, customer behavior, and even broader economic indicators, companies can use predictive models to forecast future sales with a higher degree of accuracy.
The data foundation for predictive sales forecasting is comprehensive and varied. Sales teams might start with internal sales performance data, examining seasonal trends, product popularity cycles, and the impact of previous marketing campaigns on sales volumes. This internal data is then enriched with external data sources, such as industry trends, competitor activities, and economic forecasts, to provide a broader context for the sales predictions.
Advanced analytics tools and machine learning algorithms play a crucial role in processing this data. These technologies can identify complex patterns and relationships within the data that might not be immediately apparent through traditional analysis methods.
4. Improving customer segmentation
Improving customer segmentation is another powerful example of data-driven decision-making in action. By analyzing detailed customer data, businesses can divide their market into distinct segments based on a variety of criteria such as demographics, purchasing behavior, preferences, and engagement levels. This refined segmentation allows for more targeted and effective marketing strategies, product development, and customer service approaches.
5. Enhancing website conversion rates
By meticulously analyzing website visitor data, companies can identify obstacles in the user journey, pinpoint areas for improvement, and implement changes that lead to higher conversion rates. This process involves a deep dive into metrics such as page views, bounce rates, navigation paths, and conversion funnels.
For instance, an e-commerce company might analyze its website data and find that a significant number of users abandon their shopping carts on the payment page. Further investigation could reveal that the checkout process is too complex or that there aren’t enough payment options available. Armed with this insight, the company decides to simplify the checkout process and add more payment methods. Post-implementation data shows a decrease in cart abandonment rates and an increase in completed purchases, directly linking the data-driven decision to a positive outcome.
Overcoming Challenges in DDDM
Data-driven decision making is a powerful approach, but it's not without its hurdles. Below, we outline common challenges and strategic solutions of DDDM.
1. Data quality and integrity
A significant challenge in data-driven decision-making is ensuring data quality and integrity. Flawed data can lead to inaccurate analyses, misguided strategies, and ultimately, suboptimal business outcomes. Issues such as incomplete datasets, inconsistencies across data sources, and outdated information are common challenges that compromise data reliability.
Solutions:
- Regular data audits: Conducting frequent audits helps identify and rectify inaccuracies, ensuring data remains current and accurate. This proactive approach aids in maintaining the high quality of data necessary for effective decision-making. Another solution is to integrate a business data QA solution to monitor and validate data at all times and notify of any anomalies and inconsistencies.
- Implementing standardized data collection processes: Establishing uniform procedures for data collection across various channels and touchpoints minimizes inconsistencies and errors, enhancing data integrity.
- Leveraging data integration tools: Solutions like Improvado play a crucial role in overcoming data quality challenges. Improvado automates the process of aggregating data from multiple sources, standardizing datasets, and ensuring that data is up-to-date and consistent. This streamlines data preparation efforts and significantly reduces the likelihood of human error, thus preserving data integrity.
2. Data silos
When data is isolated within different departments or systems within an organization, it hampers the ability to gain a holistic view of business operations and customer interactions. This compartmentalization leads to disjointed analyses, inefficiencies, and missed opportunities for synergy across business functions.
Solutions:
- Adopting integrated data management platforms: Implementing a unified platform that consolidates data from various silos enables a comprehensive analysis. This approach ensures all decision-making is based on a complete dataset, offering a more accurate and holistic insight into business performance.
- Promoting cross-departmental collaboration: Encouraging collaboration between departments helps break down silos. Sharing data and insights across teams fosters a unified strategy that leverages diverse data sources for a more rounded decision-making process. For instance, sales and marketing alignment has proven to help B2B and B2C organizations close 38% more deals and generate up to 208% more revenue from their marketing efforts.
- Utilizing data integration tools: Tools like Improvado are instrumental in dismantling data silos. Improvado efficiently aggregates data from disparate sources into a centralized system, automating the integration process. This not only simplifies access to and analysis of data across the organization but also ensures that decisions are informed by a comprehensive understanding of all relevant information.
- Establishing data governance policies: Clear data governance strategies ensure that data across the organization is standardized, accessible, and secure. This fosters an environment where data is shared and utilized effectively, countering the fragmentation caused by silos.
3. Analysis Paralysis
Analysis paralysis is a notable challenge in data-driven decision-making. It occurs when the sheer volume and complexity of data available overwhelm decision-makers, leading to delayed actions or indecision.
Solutions:
- Prioritizing relevant data: Focus on data that directly impacts decision-making. Not all data is equally important; prioritizing data based on relevance to specific objectives can significantly reduce the burden of analysis.
- Setting clear objectives: Having well-defined goals helps to narrow down the scope of analysis, ensuring that efforts are concentrated on gathering and interpreting data that is most pertinent to achieving these objectives.
- Leveraging simplified analytical tools: Utilizing tools that streamline the analytical process can help cut through the complexity. Platforms like Improvado AI Agent offer a simple way to base all your decisions in data — simply ask AI. AI Agent is connected to your marketing dataset and can query data based on your questions written in plain language.
- Fostering a decisive culture: Cultivating a company culture that values decisiveness and encourages taking calculated risks can mitigate the effects of analysis paralysis.
- Implementing incremental decision-making: Adopting an approach where decisions are made in stages can help manage the overwhelm. This involves making preliminary decisions based on available data, then refining those decisions as more data becomes available or as outcomes are evaluated.
4. Measuring the ROI of Data Initiatives
A significant challenge in data-driven decision-making is quantifying the return on investment (ROI) of data initiatives. This difficulty stems from the often indirect impact data projects have on revenue and the complexity of attributing financial outcomes to data-driven insights and actions.
Solutions:
- Establish clear KPIs: Before launching a data initiative, define specific, measurable key performance indicators that align with the expected outcomes of the project. This allows for a more direct assessment of its impact.
- Implement a phased approach: Break down the data initiative into smaller, manageable phases with specific goals and metrics. This not only makes the project more manageable but also allows for interim evaluations of effectiveness and ROI.
- Conduct regular reviews: Schedule periodic reviews of data initiatives to assess their performance against the set KPIs. This continuous evaluation helps in making adjustments to strategies based on what is or isn't working, thereby optimizing the ROI.
A Solid Data Foundation for Data-Driven Decision-Making
Improvado offers solutions to streamline data integration, processing, and analysis, enabling businesses to focus on strategic decision-making rather than data management. As we've explored, the benefits of adopting a data-driven approach are clear: enhanced accuracy in decision-making, improved operational efficiency, and a competitive edge in the marketplace.
Book a demo call to unlock new opportunities for growth and innovation through data-driven decision-making.
Frequently Asked Questions
What is data-driven decision making (DDDM)?
Why is data-driven decision-making important for businesses?
How can businesses implement DDDM?
What are common challenges in data-driven decision-making?
How does DDDM impact marketing and sales?
500+ data sources under one roof to drive business growth. 👇
Achieve 99.9% data accuracy & 80% faster reporting like our clients