AI Marketing Campaigns: The Future of Advertising in 2024
In 2023, artificial intelligence has made a huge leap, and marketing is gaining the most out of it. AI is enhancing how brands understand and meet customer needs, moving beyond traditional methods. This shift towards AI-driven methods is reshaping the way companies approach marketing campaigns, bringing in new avenues for creative, personalized, and impactful advertising campaigns that resonate deeply with audiences. This guide explores the key aspects of leveraging AI for marketing campaigns ideation and execution.
What Is an AI Marketing Campaign?
Here's a good illustration of how to apply AI to marketing campaigns, from demand generation to customer re-engagement.
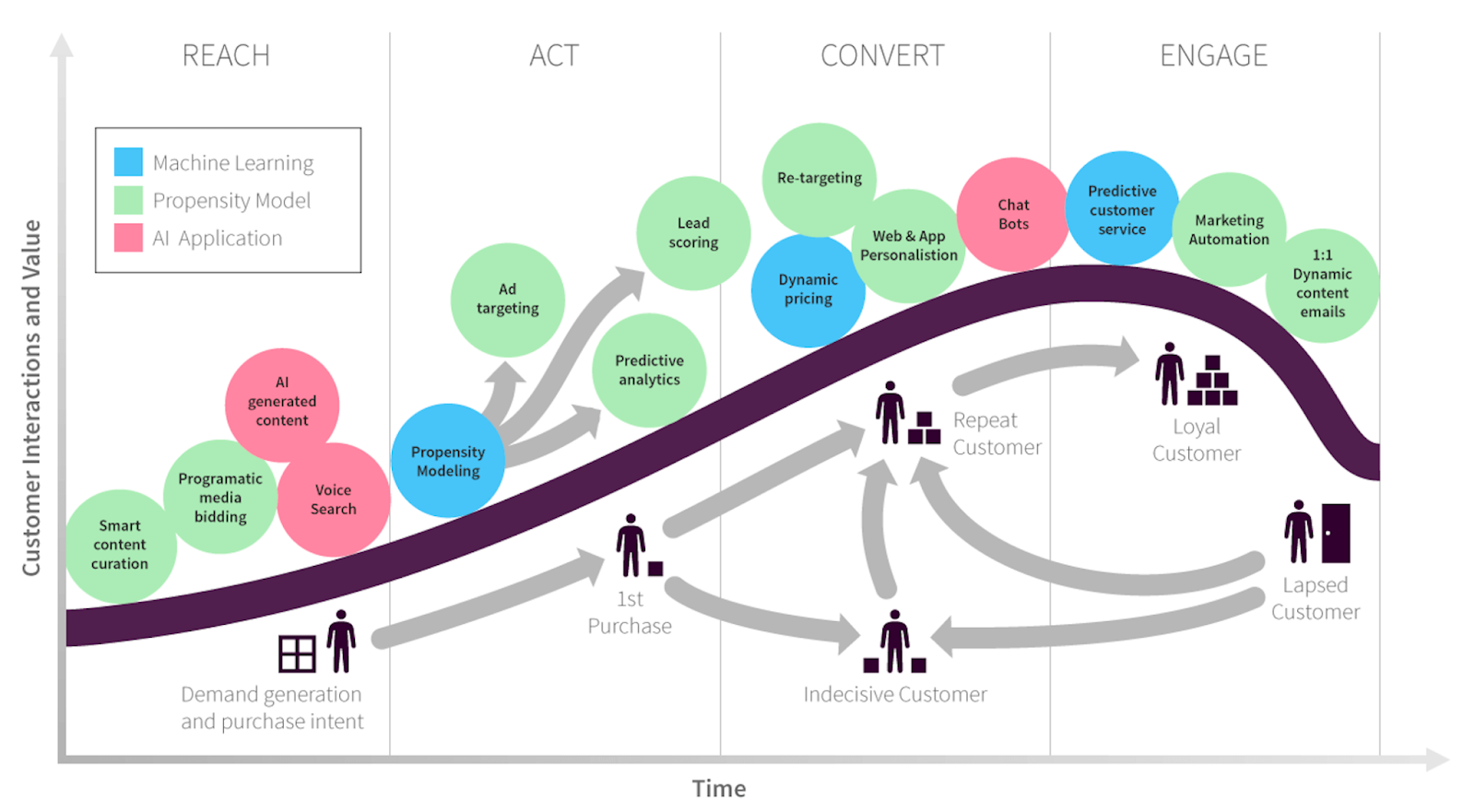
AI for Marketing Campaigns: A Deep Dive into Techniques
AI isn't just one tool, but a collection of advanced technologies, each bringing unique strengths to the table. Let's explore the diverse range of AI techniques that are currently driving AI ad campaigns.
Machine Learning (ML): The Precision Tool
The core functionality of ML in innovative marketing campaigns
- Pattern recognition: ML algorithms are adept at sifting through extensive datasets to detect recurring patterns, trends, and anomalies. This capability is crucial for understanding customer behaviors and preferences, and predicting future actions.
- Target audience segmentation: By analyzing customer data, ML helps segment audiences more accurately, enabling marketers to tailor campaigns to specific groups based on demographics, behaviors, and purchase histories.
- Optimized budget allocation: ML can analyze past campaign performances to inform future budget distribution, ensuring resources are allocated to the most effective channels and strategies.
- Dynamic content personalization: Leveraging ML, marketers can personalize content at scale. From email marketing to online ads, content can be dynamically adjusted to resonate with individual preferences, significantly enhancing engagement and conversion rates.
- Performance forecasting: ML algorithms can project the future performance of marketing campaigns, helping marketers anticipate outcomes and make proactive adjustments.
Natural Language Processing (NLP): Bridging the Communication Gap
How to use NLP in AI campaigns
- Understanding customer sentiment: NLP excels in sentiment analysis, parsing customer feedback, social media conversations, and online reviews to gauge public sentiment toward brands, products, or campaigns. This understanding aids marketers in fine-tuning messaging and addressing customer concerns proactively.
- Content optimization: NLP tools can optimize content for SEO, ensuring it resonates with target audiences and search engine algorithms. By analyzing keyword trends and search behaviors, these tools help in crafting content that increases visibility and engagement.
- Voice search optimization: As voice search becomes more prevalent, NLP is pivotal in optimizing content for this medium. It ensures that verbal queries align with the content, improving a brand’s visibility in voice search results.
Large Language Models (LLM): Chatting with Your Data
LLMs and AI ad campaigns
One of the most significant advantages of LLMs in marketing campaigns is their ability to facilitate a direct conversation with data. Marketers can input queries in natural language and receive insights in a format that is easy to understand and act upon.
Improvado AI Agent is an example of a marketing analytics solution powered by a custom large language model. AI Agent enables users to interact with marketing data in natural language, with the help of a chat-like interface. The agent will translates your questions into SQL and queries your dataset to provide you with an answer. It's like a front-end for your dataset that simplifies access to your data and breaks down the barriers often presented by traditional data analysis methods that require technical expertise and take a lot of time
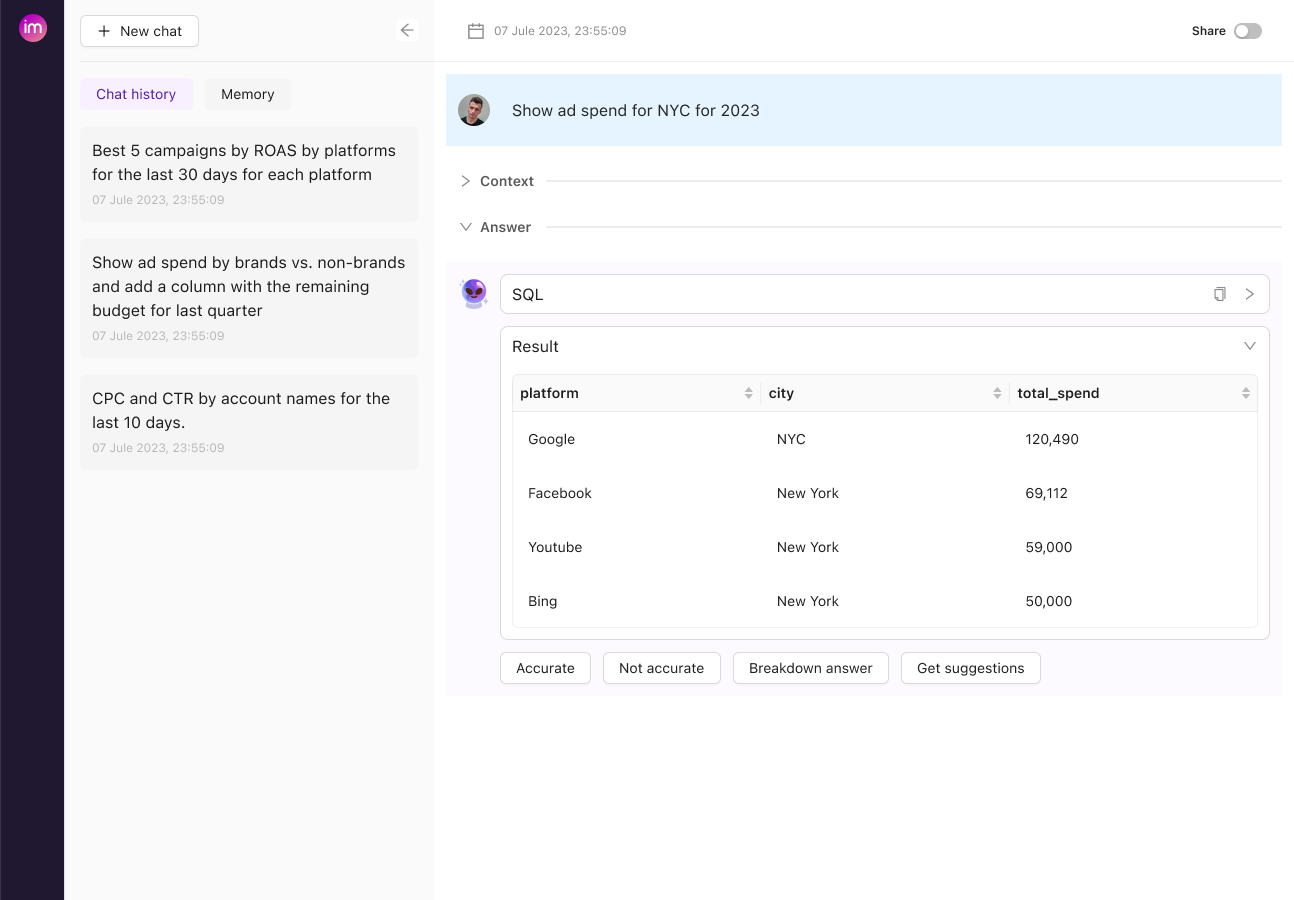
How to use AI Agent to run marketing campaigns:
- Track campaign performance: AI Agent can ask any questions about the performance of your campaigns. Marketers who are already using Improvado AI Agent often query something along the lines of ‘Show me the daily combined ad spend from Google and Bing over the past week’ or ‘Show me the top 5 campaigns that generated the highest ROI in the current quarter.’
- Optimize allocation of resources: Instead of logging in to multiple platforms and analyzing the data, ask AI Agent to evaluate the performance of campaigns based on ROAS, and analyze metrics like CPC and CTR for individual account names and platforms. By identifying which channels perform best, you can allocate budgets and resources more effectively, maximizing ROI.
- Monitor budget pacing: AI Agent enables comparison of ad spend for varied categories, assessing ad spend against the remaining budget for distinct timeframes, such as quarterly or annually.
- Develop naming conventions: Simply ask the Agent to write naming convention rules based on the campaign names you have.
These are only some examples of how to apply AI Agent in your marketing operations. To put it simply, if you have a question, AI Agent will present you with an answer.
Image Recognition: The Visual Scanner
Striving for the best AI advertising campaigns with image vision
Image vision helps to analyze marketing campaigns on a more granular level that is often overlooked and do it at scale. Here is a couple of examples of how to apply AI image vision:
- Analyze the influence of color on consumer behavior: AI image vision can analyze the visual elements of an advertising campaign, such as color schemes, imagery, and branding elements like logos or product placement. This analysis can reveal how these elements contribute to the campaign's overall performance. For instance, it might find that certain colors or images resonate more effectively with the target audience, leading to higher engagement rates.
- Monitor brand visibility across various platforms: This helps marketers understand the reach and impact of their visual branding efforts and can inform decisions about future ad placements and brand partnerships.
- Leverage AI for contextual Ad placement: By analyzing the content and context of potential ad spaces, AI ensures that ads are placed in the most relevant and engaging environments. This targeted approach helps in maximizing ad effectiveness and audience relevance, potentially leading to better conversion rates and ROI.
- Real-time ad performance optimization: AI image vision can provide immediate feedback on how certain visual elements are performing, allowing marketers to make data-driven adjustments. This could involve tweaking visual elements, changing ad placements, or altering the campaign's visual messaging to better align with audience preferences.
AI Image Generation: From Text to Visual in Minutes
<p class="c-block-quote-embed-05">AI image generation technology, also known as text-to-image generation, is a pretty self-explanatory concept. This technology typically involves the use of advanced machine learning models that can create visual content based on textual input.</p>
The core use cases of the image generation technology in AI ad campaign
Text-to-image technology is changing the way marketers visualize ideas. AI image generation bypasses traditional methods of graphic design, enabling marketers to quickly create visual content directly from campaign ideas or textual content.
It streamlines the creative process, allowing for the rapid production of images for social media posts, digital ads, or website graphics. Marketers can input campaign-specific keywords or descriptive phrases called AI image prompts, and the AI generates tailored images that align with the campaign's theme and tone.
AI image generation aids in the A/B testing of visual content. Marketers can quickly produce multiple variations of an image to test which visual resonates best with their target audience. This rapid iteration process can significantly improve engagement rates and overall campaign effectiveness.
Overall, AI image generation addresses the challenges of time constraints and budget limitations. With this technology, the need for extensive graphic design resources is reduced.
Here's a real-life example of an ad campaign with visuals generated entirely by artificial intelligence. Heinz used the image generator DALL-E 2 to launch its Heinz A.I. Ketchup campaign. The company even used user-suggested prompts and visuals and distributed them across social posts and print ads.
The Nutella case shows how AI image generators help launch campaigns at an outstanding scale. The company leveraged AI image generation technology to come up with 7 million unique Nutella jar designs. The label design algorithm completed the task that would otherwise require an army of designers.
Deep Learning: Unearthing Deeper Insights
How to use deep learning in AI marketing campaigns
- Analyzing customer behavior: Use deep learning technology to analyze data from multiple sources, including social media interactions, website traffic, and purchase history. By processing this information, it identifies patterns and trends that might be invisible to conventional analysis methods. Marketers can leverage these insights to tailor their campaigns more effectively, ensuring that they resonate with their target audience.
- Predictive modeling: By analyzing past campaign data, deep learning tools can predict future consumer responses and buying patterns. This foresight allows marketers to adjust their strategies proactively, focusing on areas with the highest potential return on investment.
- Optimizing ad campaigns: Deep Learning algorithms can optimize bidding strategies and ad placements in real-time. By constantly analyzing campaign performance data, they adjust bids and placements to maximize visibility and conversion rates. This real-time optimization ensures that ad budgets are utilized effectively, yielding better results.
- Enhancing creative content: Deep learning aids in creating more impactful creative content. By analyzing successful elements in past campaigns, it can guide the creative process, suggesting themes, colors, and imagery that are more likely to engage the target audience.
Predictive Analytics: Forecasting the Future
How to apply predictive analytics for ad campaign success
Predictive analytics in AI marketing campaigns offers a data-driven approach to planning and executing marketing strategies:
- Campaign result prediction: Predictive analytics allows marketers to estimate the potential success of a campaign before it even launches. By analyzing past campaign data, AI can predict customer responses, conversion rates, and potential revenue, enabling marketers to make informed decisions and allocate resources more effectively.
- Customer behavior forecasting: Predictive analytics can analyze past buying patterns and engagement data to forecast future customer actions, such as likelihood of purchase, product preferences, and potential churn. This insight is invaluable for targeting and personalizing marketing efforts.
- Budget optimization: By predicting which channels and tactics are likely to yield the best results, marketers can optimize their spend, reducing waste and increasing ROI.
- Lead scoring and prioritization: Not all leads are created equal. Predictive analytics can score leads based on their likelihood to convert, helping marketing teams prioritize their efforts and tailor their approach to each segment.
- Ad content and placement optimization: By analyzing which types of ad content and placements have historically performed best, predictive analytics can guide marketers on how to craft and place future ads for maximum impact.
Volkswagen has been using artificial intelligence in its media buying strategies in Germany for a while, with significant success. The use of AI recommendations has led to higher car sales compared to those achieved through traditional media agency recommendations. The brand saw a 14% increase in dealership orders for a particular model using AI recommendations.
Semantic Analysis: Understanding Context
How to apply AI-powered sentiment analysis to campaign analysis
- Content relevance and optimization: For content to resonate with the target audience, it needs to align with their interests and search intents. Semantic analysis aids in crafting content that aligns with these aspects, enhancing relevance and engagement.
- Ad targeting precision: In advertising, the context in which a message is placed can significantly impact its reception. Semantic analysis helps in placing ads in the most contextually relevant environments, ensuring they reach the audience when they are most receptive to the message. This increases ad effectiveness and drives better campaign results.
- Automated content curation: By understanding the semantics of different content pieces, AI can automate the process of content curation for marketing purposes. This ensures that only the most relevant and contextually appropriate content is selected for campaigns, saving time and enhancing campaign quality.
- Brand monitoring and reputation management: Semantic analysis is crucial for monitoring how a brand is perceived across digital platforms. It helps identify not just where the brand is mentioned but also the context of these mentions, allowing for a more proactive approach to reputation management and brand image cultivation.
Conclusion
As we conclude our exploration of AI in marketing campaigns, it's clear that these technologies are not just futuristic concepts but practical tools. And AI isn't just one tool but many advanced technologies. From machine learning refining customer segmentation to predictive analytics forecasting campaign success, AI is empowering marketers with deeper insights and more effective strategies.
500+ data sources under one roof to drive business growth. 👇
Gain marketing insights by chatting with your data in plain English