Next-Gen Marketing: Best AI Marketing Automation Use Cases
Digital marketers are facing a rapid evolution driven by AI. Tasks that once took days—like campaign reporting, performance monitoring, and complex data modeling—can now be automated and executed in minutes.
In this new era, AI marketing automation software is pushing teams to "grow up" faster, demanding greater agility, smarter decision-making, and tighter workflows.
This article explores how AI marketing automation reshapes key areas of marketing, from streamlining reporting to reverse-engineering data models, and why adopting these tools is no longer optional but a necessity for staying competitive.
What Is the Role of AI in Marketing Automation?
First, let’s define what marketing automation is and what it is not.
At its core, marketing automation is the use of technology to manage and execute marketing processes and campaigns across multiple channels, all while minimizing manual effort. It’s not a one-size-fits-all solution or a fully hands-off tool—it’s a framework that helps marketers efficiently deliver the right message to the right audience at the right time, based on predefined criteria and data-driven insights.
Despite perceived complexity, traditional marketing automation is pretty straightforward. Its rule-based approach dictates that if X, Y, Z happens, then marketing tasks like scheduling campaigns, segmenting audiences, and sending email campaigns should be completed.
However, traditional approaches require frequent monitoring and updates from marketers to remain effective over time.
By becoming more independent, AI marketing automation reduces the need for constant human oversight. Its ability to handle complex, data-driven decisions with minimal intervention allows brands to scale operations and respond to changes with unprecedented agility and precision.
Reasons Why Marketers Should Use AI Marketing Automation
Marketers have a variety of compelling reasons to embrace AI marketing automation. Some benefits directly impact revenue and cost savings, while others enhance operational efficiency, customer experience and retention.
Below, we explore the key drivers behind adopting AI in marketing workflows.
1. Economic impact and cost efficiency
According to a McKinsey report, generative AI has the potential to increase marketing productivity by 5–15% of total marketing spending.
By automating repetitive tasks, optimizing workflows, and enhancing decision-making, artificial intelligence automation allows businesses to achieve more with fewer resources. This translates into significant cost savings while driving better results.
2. Increased team productivity
AI significantly enhances team productivity by automating repetitive and time-intensive tasks such as data analysis, campaign reporting, and customer segmentation.
3. Faster speed to market
With AI tools automating data analysis, campaign adjustments, and content creation, marketers can bring campaigns to market up to 75% faster.
How exactly? For complex, multi-brand, and multi-regional campaigns, AI simplifies the coordination of numerous moving parts.
These are the three key reasons for adopting AI marketing automation.
Beyond these, AI enhances decision-making by rapidly processing vast amounts of data to uncover actionable insights that would be time-intensive for human teams. This ability to sift through large datasets is what drives real-time optimization, enabling marketers to adjust campaigns instantly, respond to shifting customer behaviors, and seize opportunities with precision.
What Are AI Agents?
Before looking into specific AI automation tools and use cases, let's understand one important concept – AI Agents.
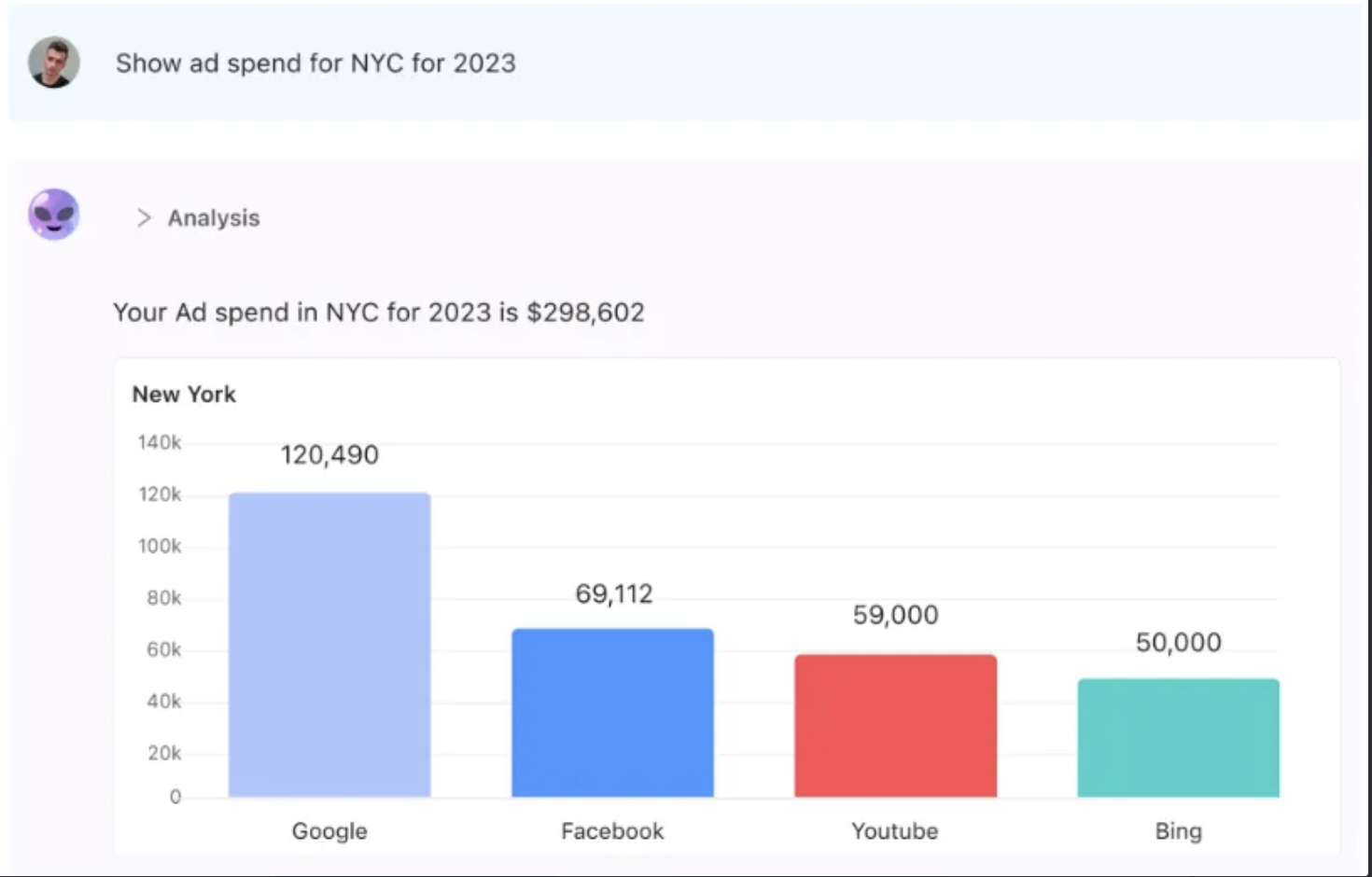
AI agents are intelligent systems designed to autonomously or semi-autonomously perform tasks based on goals or commands from users.
AI agents combine the power of natural language processing (NLP) with code execution, external data sources, and user interfaces to automate and execute workflows. By acting as intelligent intermediaries, they can interpret tasks, process data, and take action across multiple systems.

Here’s a schematic representation of how the AI agents operate.
A typical AI agent has:
- AI agent rules that define the role it plays and what expertise it has. In our case, let’s say it’s marketing analytics.
- And company rules that include knowledge of your marketing specifics and data architecture. This data turns generic AI algorithms into a tool tailored to your business and needs.
APIs play a central role in enabling AI agents to "talk" to various tools and platforms, allowing them to ingest data, send instructions, and interact across a company’s tech stack. Agents can even generate small software programs to execute tasks.
For marketers, this unlocks opportunities to automate complex, multi-platform tasks—like integrating large datasets, scaling audience building, lead scoring, or optimizing campaigns in real time—without manual intervention.
9 Applications of AI in Marketing Automation
The majority of marketers are still limiting the use of AI to content production and optimization. By introducing AI marketing automation tools into other parts of their operations, businesses can streamline processes, scale efforts, and uncover new opportunities for growth.
In the following section, we’ll dive into the 9 best AI marketing automation use cases and how they can transform the way brands operate.
1. Goal-based data extraction and loading
AI-driven goal-based data extraction and loading revolutionizes how businesses handle API integrations.
With an AI Agent, you can request a new data source connector through a simple command or click, eliminating the need for complex manual integration efforts.
The process is seamless:
- The AI agent reviews the data source’s API documentation;
- Identifies feasible solutions and implements the integration using low-code extraction services;
- Once ready, the agent validates the connection against the API.
In moments, your system gains a fully functional API connector tailored to your needs.
2. Detect and fix naming conventions anomalies
AI marketing automation tools can play a pivotal role in maintaining data accuracy and consistency by automating the detection and correction of naming anomalies within marketing campaigns.

By analyzing existing campaign datasets, AI agents can establish a clear taxonomy for naming conventions based on recurring patterns, metadata, and best practices. This taxonomy becomes the foundation for standardized naming structures across platforms, ensuring alignment.
Once the baseline taxonomy is in place, AI agents can:
- Detect anomalies: Identify irregularities such as typos, missing elements, or misformatted names in campaign assets, ad groups, or UTM parameters.
- Fix errors in real-time: Automatically flag or correct anomalies to bring naming conventions back in line with the approved taxonomy.
- Scale across platforms: Monitor multiple campaigns across diverse ad platforms, CRM systems, and marketing tools to ensure naming consistency at scale.
3. Monitor campaign performance and pace metrics and KPIs
Another use case of AI-powered marketing automation is tracking metrics and KPIs in real-time across all channels and platforms. AI tools provide flexible solutions for monitoring performance, from on-demand insights to automated reporting and advanced governance platforms.
1. On-demand performance insights
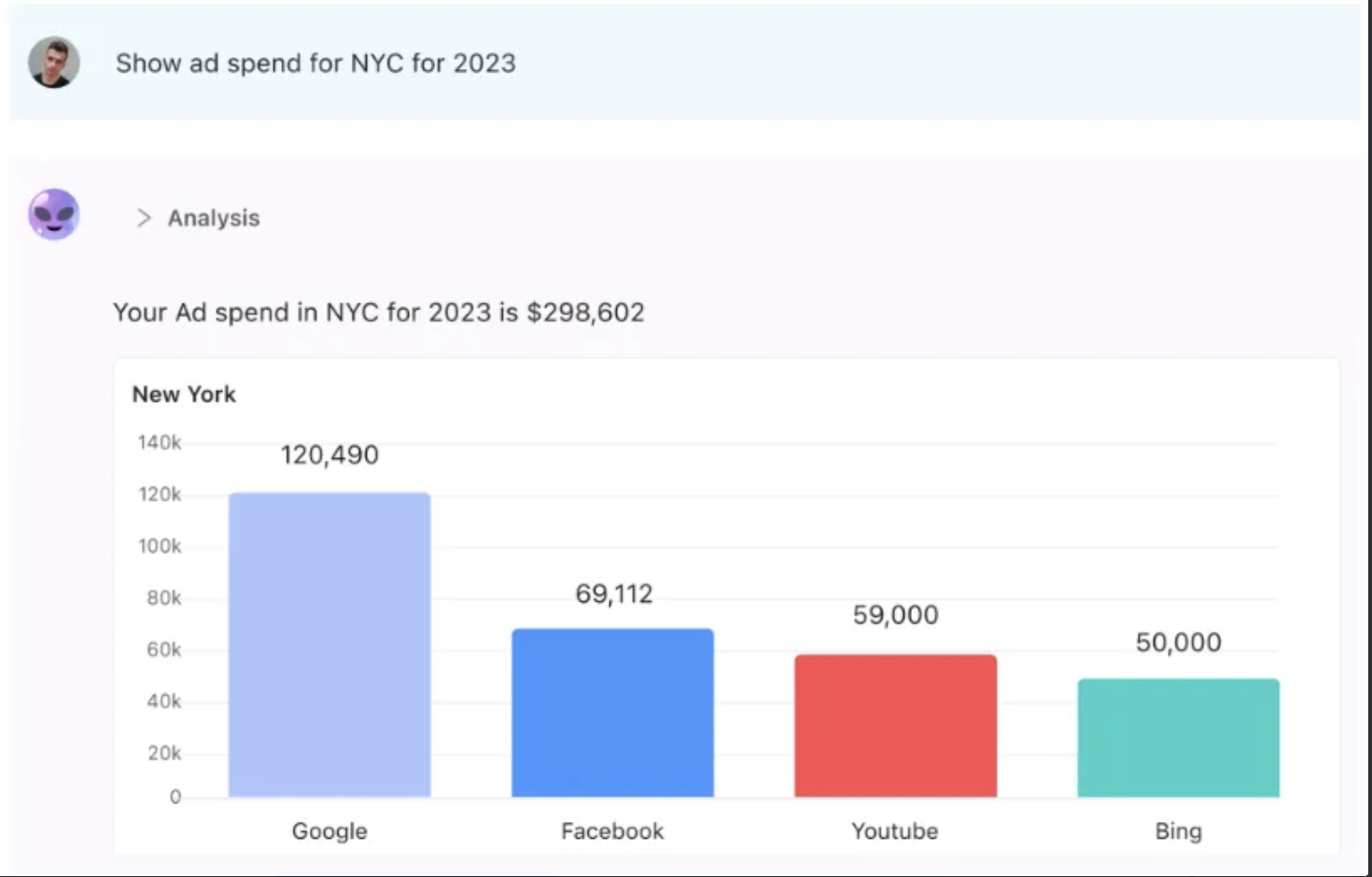
By simply asking the agent in plain language (e.g., “What are the current conversion rates for Campaign X?”), marketers can instantly access granular insights without diving into dashboards or waiting for analysts. This flexibility enables quick decision-making, particularly for fast-moving campaigns.
2. Automated period-over-period reports
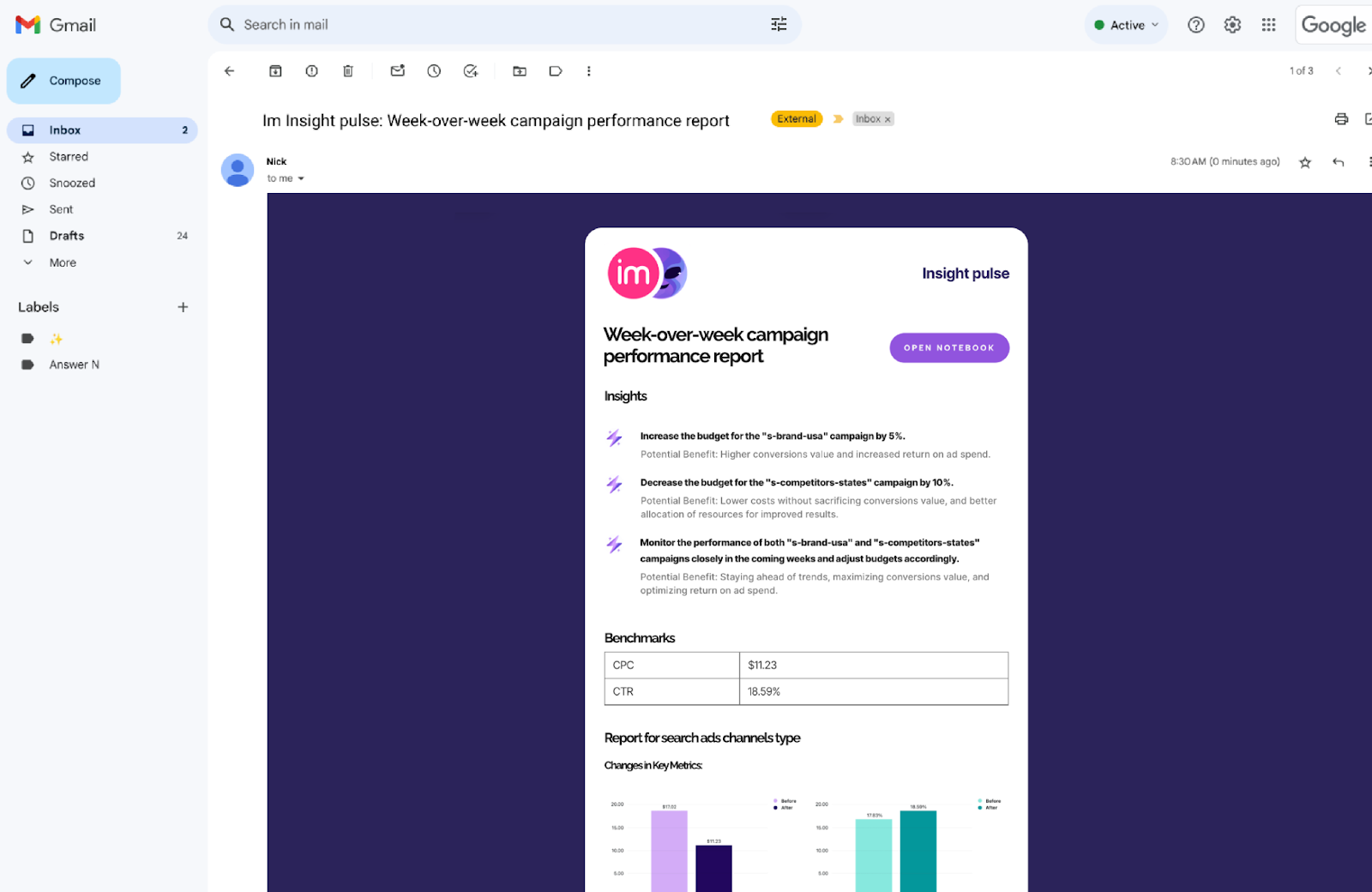
AI agents can be configured to send automated period-over-period reports (e.g., daily, weekly, or monthly) directly to your inbox. These reports highlight performance trends, compare metrics over time, and surface deviations, ensuring stakeholders are updated without manual effort. This saves time while ensuring no critical KPIs are overlooked.
3. AI-powered tools for metric pacing

Marketing Data Governance enables real-time monitoring and adjustments of campaigns against performance benchmarks and metrics
Advanced AI tools, such as Marketing Data Governance, monitor pacing metrics across multiple platforms and campaigns. By automating cross-channel tracking, Marketing Data Governance:
- Comparse spend and performance against targets to detect over- or under-pacing.
- Flags anomalies, such as sudden drops in impressions, clicks, or conversions.
- Ensures budgets and KPIs are aligned with business goals in real time.
4. Complex data modeling
Traditional data modeling often requires extensive manual effort to clean, organize, and connect data points, especially when dealing with multiple platforms and data sources.
AI tools for marketing automation can simplify complex data modeling by streamlining the mapping, transformation, and alignment of large datasets for specific use cases.
AI agents can automatically ingest data from various sources (e.g., ad platforms, CRMs, and analytics tools) and map it to a predefined structure or taxonomy tailored to the business’s needs.
For example, if a marketer needs to analyze customer lifetime value (CLV) or ROAS across campaigns, AI agents can:
- Transform raw data into clean, analysis-ready formats.
- Map disparate data points to create a unified model, ensuring all metrics align (e.g., matching campaign spend to customer acquisition data).
- Apply business-specific logic, such as custom attribution models or multi-touch conversion paths, to generate actionable insights.
For advanced use cases, AI can also build predictive models that forecast trends or outcomes, like customer churn, campaign effectiveness, or optimal budget allocation. This enables marketing teams to move beyond descriptive analytics and adopt a forward-looking, data-driven approach.
5. Reverse-engineer models
Building analytical dashboards often requires months of design, iteration, and deployment.
Traditional workflows start with raw data, which is modeled, cleaned, and aligned to produce dashboards. AI marketing automation accelerates this process and introduces a reverse-engineering capability: working from dashboards back to the underlying data.
AI agents can quickly analyze existing dashboards to identify the structure, relationships, data sources and metrics in use. They then generate analysis-ready datasets that can be plugged into BI tools or reporting platforms for immediate use.
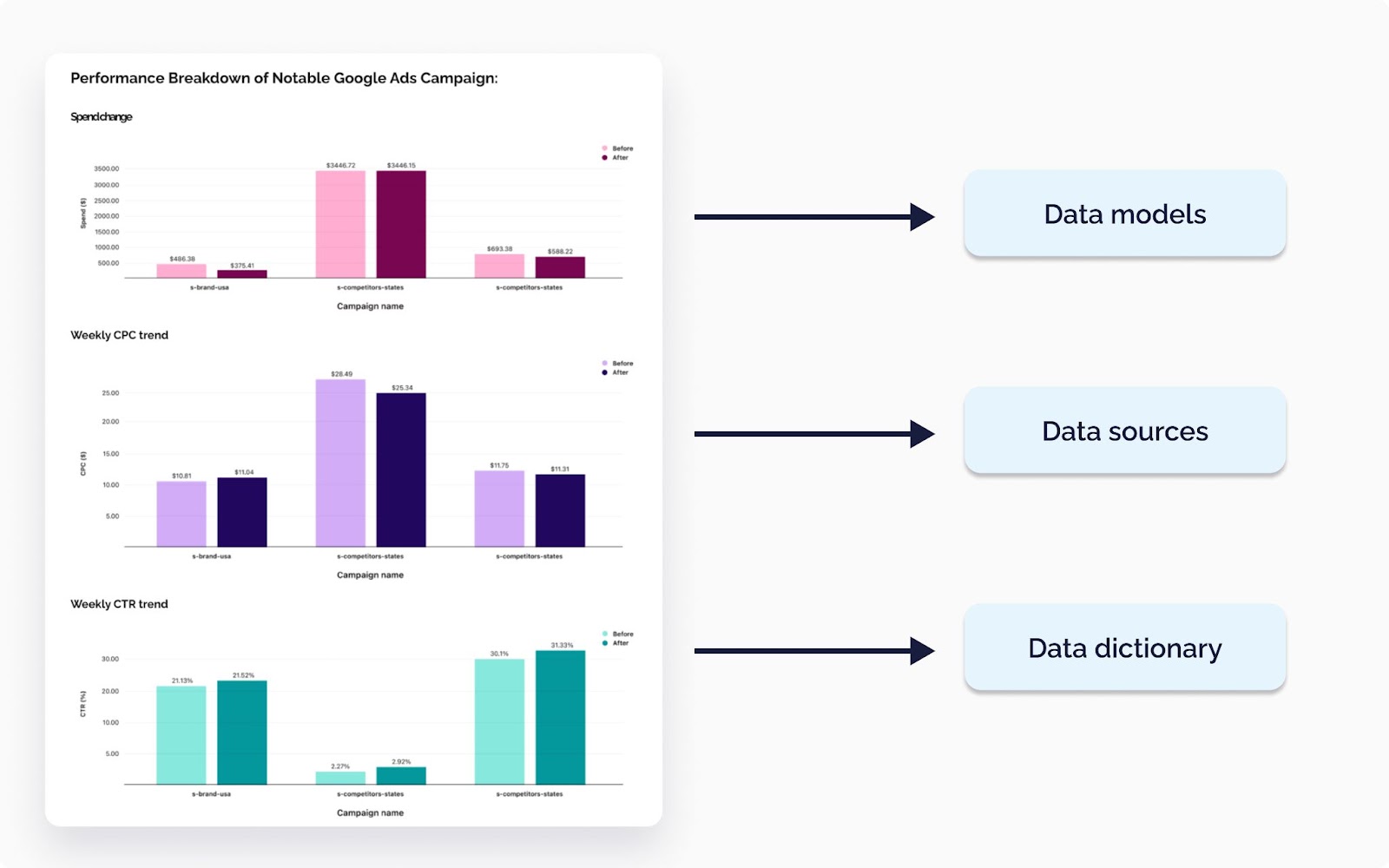
In the reverse process, AI can deconstruct dashboards to trace key metrics back to their raw data sources. For instance:
- If a dashboard tracks campaign ROAS, the AI agent identifies the spend, conversion, and revenue data that feed into the calculation.
- The AI validates each component, flags missing data, and recreates the analytical pipeline as needed.
6. Design and build reports from prompts
Marketing reporting often lags behind real-time needs, with daily or weekly reports arriving days late—leaving teams reactive instead of proactive.
AI marketing automation tools address this bottleneck by designing and delivering reports on demand in minutes, not days.

AI agents can generate reports from simple, natural language prompts. For example, a user can ask, “Show me campaign performance for Q4, broken down by channel and region,” and the agent dynamically pulls, processes, and visualizes the data.
Beyond ad-hoc requests, AI agents can automate recurring reports with minimal setup.
Once configured, they fetch the latest data, apply transformations, and deliver clean, actionable insights to stakeholders—whether in dashboards, PDF summaries, or scheduled emails.
7. Ad-hoc reporting
Up to 50% of an analytics team’s time is spent on ad-hoc requests, AI automation helps analysts gain some of this time back.
Traditional reporting workflows require manual queries, collaboration with analysts, or sifting through dashboards—resulting in delays and inefficiencies.

AI marketing automation tools streamline ad-hoc reporting by enabling on-demand insights through natural language prompts. Marketers can ask ad-hoc questions like:
- What’s the ROI of my latest Facebook campaign?
- How are we pacing against monthly spend targets?
- Which product category saw the highest growth last week?
The AI agent pulls data from relevant sources, runs calculations, and delivers precise answers within seconds—without the need for SQL queries or technical expertise.
8. Predictive analytics
In large organizations running 50-100 campaigns simultaneously, keeping up with performance tracking and optimization is a significant challenge. AI marketing automation simplifies this by delivering predictive insights that enable proactive decision-making at scale.
AI analytics tools, whether it’s an AI agent or other platform, digest vast amounts of historical and real-time data to identify trends, correlations, and performance patterns across campaigns. By leveraging machine learning models, they forecast key metrics like ROI, conversions, and engagement rates, helping marketers predict which campaigns are likely to perform best and where adjustments are needed.
For example, an AI agent can flag underperforming campaigns before budget is wasted or highlight high-performing channels to allocate resources more efficiently. These insights enable teams to pivot strategies quickly, reducing lag time and ensuring that opportunities for optimization are not missed.
By automating predictive analytics, AI platforms allow marketing teams to shift from reactive monitoring to proactive campaign management, improving outcomes without adding manual effort.
9. Take actions on data finding
Marketing teams often face a gap between identifying performance issues and taking corrective action. AI marketing automation tools bridge this gap by executing predefined actions based on data findings, reducing delays and manual intervention.

For example, if budget pacing metrics reveal that a campaign is overspending, you can instruct an AI agent to automatically pause campaigns exceeding limits or reallocate budgets to higher-performing ones. Similarly, AI agents can adjust bids, update creative assets, or tweak targeting based on performance anomalies, all within a user-defined scope.
This capability transforms AI agents into autonomous assistants that act on insights in real-time. By automating responses to data findings, marketers can maintain tighter control, improve efficiency, and minimize the risk of revenue leakage.
Tips for Successfully Implementing AI Marketing Automation
Implementing AI marketing automation can significantly streamline workflows and boost efficiency, but its success hinges on proper planning and execution. Here are key considerations to ensure a smooth implementation process.
1. Ensure data readiness
“You can’t have an AI strategy without a data strategy.”
At the foundation of any effective AI marketing automation strategy is data readiness. Without clean, consistent, and accessible data, AI tools cannot deliver accurate insights or automation.
This begins with establishing a universal data layer—a centralized hub that aggregates and harmonizes data from all platforms and data sources in your marketing stack.
This layer consolidates data from CRMs, ad platforms, ecommerce systems, and analytics tools, transforming fragmented information into a single source of truth. Once unified, the data becomes easily accessible for AI marketing tools and agents to analyze, model, and act on.
2. Start small and scale gradually
Instead of overhauling entire systems, begin with targeted use cases, such as automating reporting or monitoring campaign performance. Once success is proven, scale AI across other areas of marketing operations.
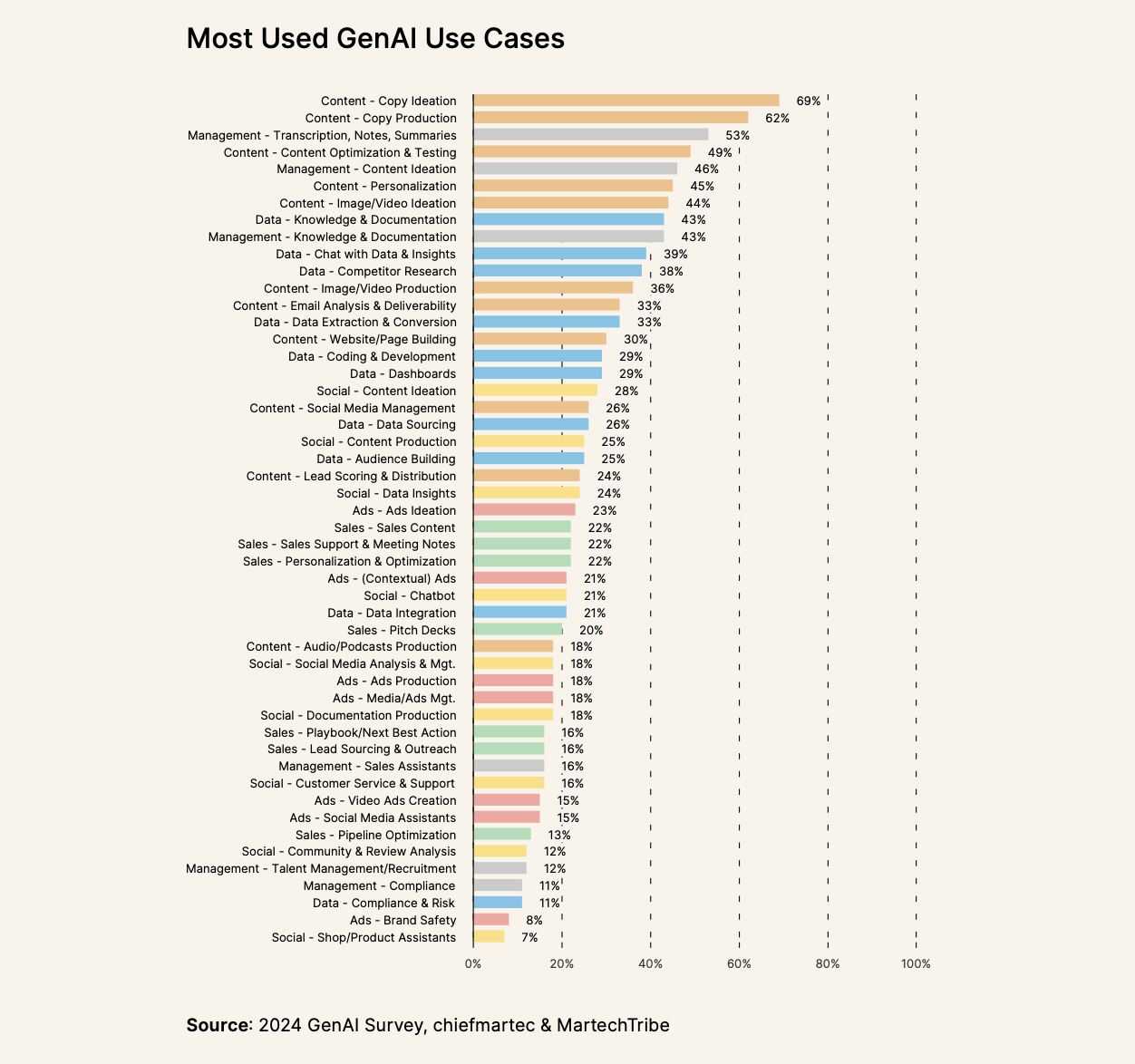
3. Invest in team training
AI tools are only as effective as the people using them.
For marketing teams, this means developing both technical and strategic skills to leverage AI for better results.
Training should focus on:
- Understanding AI capabilities: Equip teams to understand what AI can automate and how to use it to maximize efficiency.
- Interpreting AI-driven insights: Help marketers analyze AI-generated insights to make informed decisions, rather than simply relying on automation outputs.
- Hands-on tool adoption: Facilitate practical, workflow-focused training on AI tools, ensuring teams can integrate them seamlessly into existing processes.
AI marketing automation requires ongoing oversight. Regularly assess its outputs, refine workflows, and incorporate feedback to improve performance. Use insights from AI tools to identify areas for further optimization.
By following this guide, your business can confidently implement AI marketing automation, ensuring it delivers tangible results while scaling efficiently across marketing operations.
FAQ
500+ data sources under one roof to drive business growth. 👇
Automate insights and ensure data accuracy with AI-driven solutions