The buzz around AI in analytics and marketing, in general, makes it seem like everyone's riding the high-tech wave, AI-ing their strategies to the nth degree. Yet, many marketers are still figuring out how to integrate AI into their daily operations.
AI marketing analytics goes beyond the surface-level tasks of analyzing data or optimizing content. Where it truly excels is in its ability to sift through vast amounts of data and provide solid, actionable insights.
This article explores what AI analytics is and provides five powerful AI-driven data analytics use cases that revolutionize how we collect, interpret, and act on marketing data to inform decision-making and drive ROI.
What is AI Marketing Analytics?
AI can be incorporated at various stages of marketing analytics:
- Data Collection: Automate the aggregation of structured and unstructured data across multiple channels, ensuring completeness and consistency.
- Data Analysis: Utilize predictive models and machine learning to identify trends, forecast outcomes, and segment audiences with precision.
- Optimization: Apply AI-driven insights to refine strategies in real-time, adjusting campaigns for maximum ROI.
- Automation and Assistance: Use AI assistants to simplify complex workflows, such as attribution modeling, A/B testing, and personalized messaging.
By integrating AI analytics into marketing operations, brands enhance their business intelligence, making analytics more dynamic, scalable, and impactful. AI empowers marketers to move from reactive decision-making to proactive strategy, ensuring a competitive edge in the competitive landscape.
5 AI Use Cases in Marketing Analytics
Let's explore five practical ways AI analytics transforms how businesses collect, analyze, and act on data. These use cases highlight how AI enhances decision-making, from analyzing content marketing to optimizing customer journeys.
1. Enhancing Customer Segmentation with Machine Learning
Applying AI, particularly machine learning (ML), to customer segmentation helps transform vast amounts of data into precise and actionable groups.
AI can identify segments based on predicted behaviors, such as the likelihood of repeat purchases or susceptibility to churn. By integrating with campaign management tools, AI enables marketers to quickly target these segments with personalized content, optimizing marketing spend by focusing on high-value or at-risk customers.
Other examples of AI-driven customer segmentation:
- Psychographic segmentation: AI can delve into the psychographic aspects of customer data, categorizing consumers by personality traits, values, interests, and lifestyles, which can be incredibly useful for crafting resonant messaging and offers.
- Event-triggered segmentation: AI systems can segment customers based on their reactions to certain events, such as product launches or major sales. This helps in understanding how different customer segments engage with specific brand activities.
- Cross-sell and upsell segmentation: AI can identify which customers are likely to be interested in additional products or upgrades, helping marketers tailor cross-sell and upsell campaigns to receptive segments.
Over time, machine learning can further refine these segments by continuously learning from campaign outcomes, leading to even more personalized customer experiences and better conversion rates.
2. Getting Insights by Simply Asking AI Agent
With AI advancements, marketing teams can now directly communicate with their data and receive insights in real-time, using natural language bots.
This conversational analytics approach provides quick answers to complex questions about market trends, customer behavior, and campaign performance without the need for deep technical expertise, SQL querying, or IT support.
Improvado AI Agent is an example of a platform that helps uncover actionable insights though dialogue with data. It's an AI-powered analytics solution connected to your dataset that answers any question you have on marketing performance and gives you tailored advice.
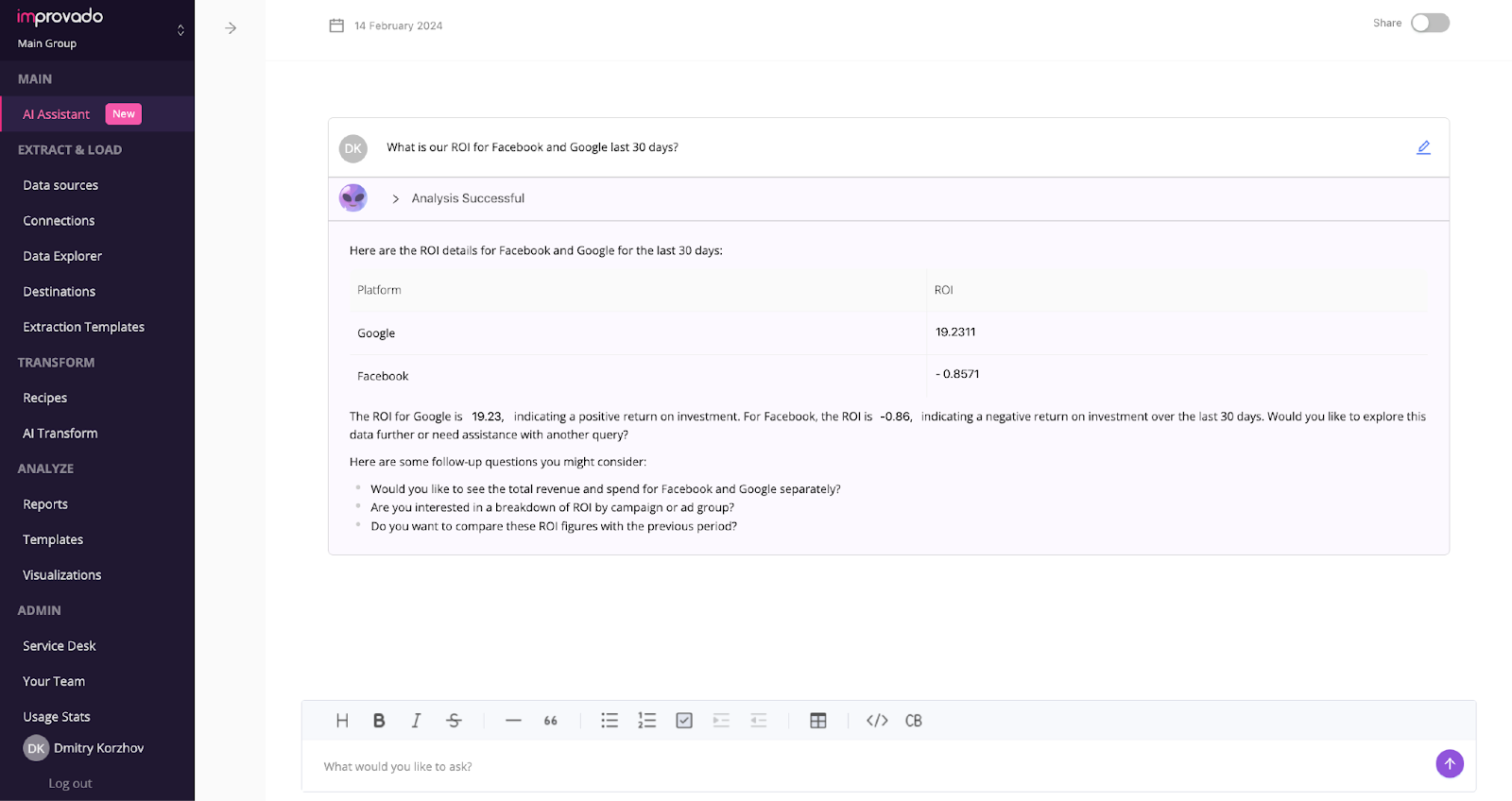
Here are some examples of analytics use cases AI Agent can handle:
- Cross-channel analytics: Use AI Agent to query ad spend across multiple platforms, evaluate the performance of campaigns based on ROAS, and analyze metrics like CPC and CTR for individual account names.
- Budget pacing: The agent can compare ad spend for varied categories, assess ad spend against the remaining budget for distinct timeframes, such as quarterly or annually.
- Data exploration & QA: You can use AI Agent to examine table updates and verify data consistency.
- Ad-hoc reporting: You can use AI Agent to address any ad-hoc analytics requests or quickly query the data and start optimizing campaigns on the fly.
How it works?
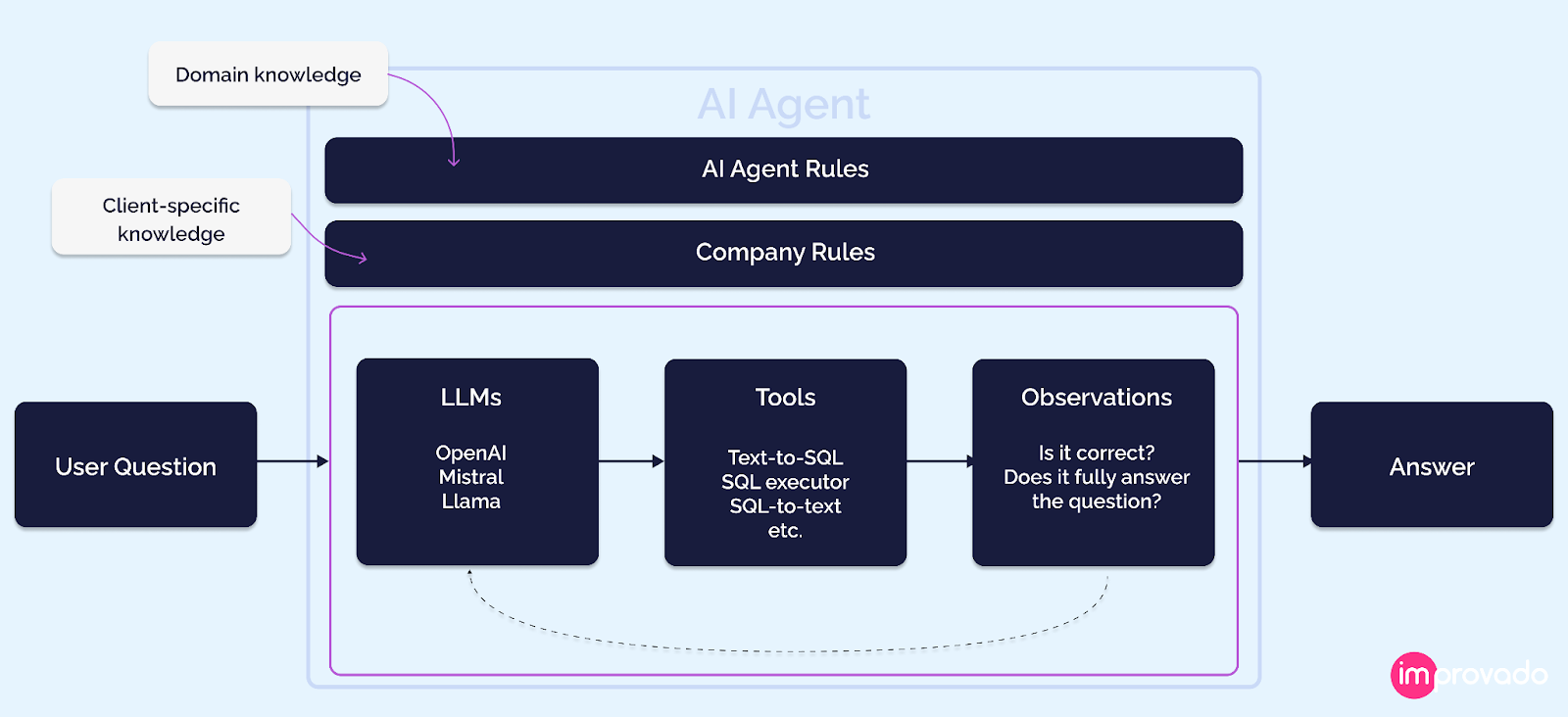
AI Agent is powered by a custom large language model (LLM) and text-to-SQL technology. You can ask any questions in plain English in a chat-like user interface, and AI Agent will translate it into SQL and query your data to provide you with findings.
Get a demo to see the full scope of AI Agent's analytics capabilities and streamline your marketing reporting.
3. Utilizing Natural Language Processing for Sentiment Analysis
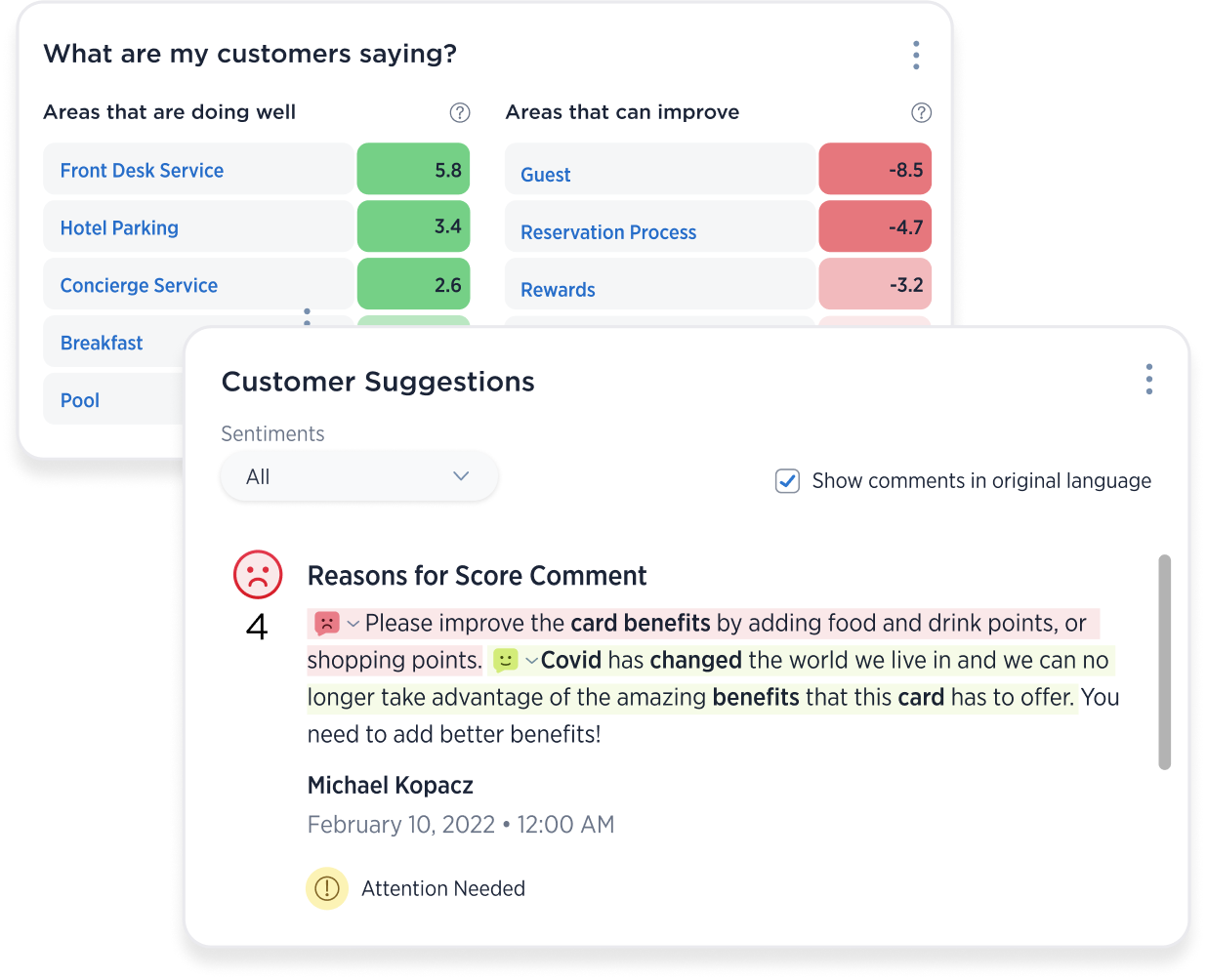
Marketers often face the challenge of deciphering customer opinions on new product launches or brand campaigns. While traditional survey methods provide direct insights, they can be time-consuming, may not capture the spontaneous sentiments expressed across digital platforms, and often show socially accepted answers.
Social sentiment analysis platforms powered by Natural Language Processing (NLP), on the other hand, can swiftly sift through large volumes of text to identify sentiment at scale. It's adept at processing data from social media, customer reviews, and discussion forums to discern general mood and opinion trends.
Marketers are now turning to Large Language Models (LLMs) like ChatGPT, with its standard chat interface, sentiment analysis plugins, and API, for enhanced sentiment analysis. Since it has been trained on a vast corpus of text data it is expected to address some of the issues of more traditional monitoring tools:
- LLMs can consider the broader context in which a statement is made, potentially leading to more accurate sentiment interpretation.
- LLMs can be fine-tuned and customized for specific industries or topics, which can improve the accuracy of sentiment analysis for niche markets or particular types of products.
- Unlike standalone tools, LLMs can be integrated into a broader AI ecosystem, combining sentiment analysis with other data sources for a more holistic understanding of customer opinions.
4. AI Image and Video Analysis for Brand Monitoring
Visual brand monitoring, the process of tracking and analyzing the use and context of a brand's visual assets across various digital platforms, has become an essential part of maintaining brand integrity. With the volume of content being shared every minute and the number of platforms a brand appears on, this task has grown beyond the capabilities of human teams alone. AI marketing analytics tools automate the detection and analysis of brand elements within visual content.
One way to do it is by using OpenAI Vision API. The Vision API is an advanced AI system that can analyze images and videos to recognize logos, products, activities, and other visual elements.
Step-by-step guide to using the vision API for brand monitoring:
- Integration: Begin by integrating the Vision API with your existing digital asset management system. This process typically involves some development work to ensure a seamless flow of data between the API and your brand’s content repositories.
- Setting parameters: Define what the API should look for in images and videos. Parameters can include your logos, product placements, brand colors, and any other visual elements that are relevant to your brand identity.
- Automated analysis: As your brand’s content spreads across various channels, the Vision API works in real-time to analyze visual elements. It detects and catalogs the presence of your brand across user-generated content, social media, and online advertising platforms.
- Actionable insights: Receive alerts and reports on where and how your brand is being represented visually. The API can spot unauthorized use of your logo, monitor the consistency of your brand portrayal, and evaluate the effectiveness of your product placements.
- Brand compliance: Use the insights to ensure that all visual representations of your brand comply with your guidelines. Quickly identify instances where the brand is used inappropriately and take action to rectify the issue.
- Competitor monitoring: You can extend the API's capabilities to keep an eye on your competitors. Track their visual presence and compare it with your brand's visibility to identify competitive insights and opportunities.
- Trend analysis: The Vision API’s analysis can highlight emerging trends in how visual content is engaging your audience. This insight can help in optimizing marketing campaigns and ensuring your creatives resonate with the target audience.
5. AI for Voice Search Optimization
The landscape of search has changed significantly. 50% of consumers in the U.S. turn to voice assistants for their queries daily. 34% use voice search weekly, and 16% monthly.
The importance of optimizing content for voice search becomes vital. This shift means moving beyond traditional keywords to a more conversational approach that aligns with natural speech patterns.
Artificial intelligence is nailing this approach. It helps analyze large volumes of voice search data to detect patterns in the way people phrase their queries. This insight is crucial for shaping content that fits seamlessly into the voice search narrative, ensuring your brand's visibility in a voice-driven world.
Here’s a couple of AI applications for voice search optimization and analysis:
- Voice search analytics: AI algorithms can analyze the tone, phrasing, and semantics of voice queries to uncover what users are really asking for. Marketers can use these insights to craft SEO strategies that resonate with the natural, informal style of spoken searches.
- Understanding voice searches with NLP: Natural Language Processing (NLP) gives AI the ability to interpret the intent and nuances of voice queries. This is crucial for creating content that speaks directly to the user, using the same conversational language that they use in their daily lives.
- Content that converses: The spoken word is naturally engaging, and AI can help create content that captures this conversational quality. By using algorithms that specialize in generating human-like text, AI tools enable marketers to produce content that feels like one side of a dialogue. This kind of content is naturally suited to voice search and can dramatically improve a brand's visibility in voice search results.
- Semantic search optimization: AI analytics solutions helps understand semantic search, where the context and relationship between words are analyzed to provide more accurate search results. By optimizing content for semantic search, marketers improve the chances of their content matching with the user's intent.
- Automated testing and optimization: AI can automate A/B testing for SEO strategies to determine what works best for voice search. This includes testing various aspects of content, from keyword density to the structure and format, to improve its discoverability via voice search.
- Classifying Search Intent with AI: AI analytics tools can be trained to classify the intent behind voice searches more effectively, distinguishing between informational, navigational, transactional, and local intents. This classification helps in creating more targeted content.
Benefits of AI in Analytics
Why should businesses bother AI-ing their marketing analytics? The integration of AI into marketing analytics not only streamlines operations but also unveils opportunities previously hidden in vast amounts of data.
- Faster insights: AI analytics solutions can sift through vast amounts of data in a fraction of the time it would take humans. This rapid analysis means businesses can gain crucial insights almost instantly.
- Informed choices: AI-driven analytics tools ensures that marketing strategies are grounded in solid data, reducing the reliance on guesswork and intuition. The result is more effective and targeted marketing campaigns.
- Personalization: AI has the capability to understand individual customer preferences. This allows for the creation of ads and promotions tailored specifically to individual tastes, leading to increased customer satisfaction.
- Cost-efficiency: Automating data analysis with AI can lead to significant cost savings. Businesses can streamline their teams dedicated to data analysis and avoid wasteful spending on ineffective marketing strategies.
- Addressing customer needs: By understanding and predicting what customers are looking for, businesses can improve their offerings, leading to a more satisfying customer experience.
- Predicting future trends: AI uses historical data to make predictions about future market trends. This foresight allows businesses to be proactive, preparing for upcoming shifts in the market.
- Streamlined operations: Streamlined operations: AI automates routine and repetitive tasks, saving hours of work. This not only speeds up processes but also allows human employees to focus on more strategic tasks.
- Minimized errors: AI systems, as machines, are less prone to the errors that can occur with human analysis, especially when dealing with vast datasets.
AI promises a future where data-driven insights reign supreme, guiding businesses towards more strategic and informed decisions. While challenges exist, with the right approach and practices, the AI revolution in marketing analytics can lead to unparalleled growth and success.
Developing an AI-Driven Marketing Strategy
Knowing all the benefits of AI in marketing analytics, the next question is how to develop an AI-driven marketing strategy and prepare your marketing department for technological transformation.
Here are 5 steps that will help you integrate AI into your operations:
1. Define clear objectives
Start by identifying specific goals AI can help achieve, such as improving customer engagement, automating campaign management, or predicting purchasing behavior. Clear objectives ensure that AI tools align with your business needs.
2. Assess data infrastructure
AI thrives on data. Evaluate whether your current data systems can handle the volume, variety, and velocity required for AI analytics. Ensure your data is clean, structured, and accessible to fully unlock the potential of AI.
Tools like Improvado streamline this process by aggregating, cleaning, and harmonizing data, providing a solid foundation for AI-driven insights while reducing manual effort. A robust data infrastructure ensures your AI models are fueled with reliable, actionable information.
3. Select the right tools
Choose AI-driven marketing tools tailored to your needs. Whether it's AI CRM tools, predictive analytics, chatbots, or generative AI, the right tools will determine the success of your strategy.
4. Train and upskill teams
Effective AI solutions require close collaboration between marketing, data science, and IT departments. Continuous dialogue is essential to move from concept to deploying practical tools that address real business challenges and deliver measurable outcomes.
But incorporating AI isn’t just about technology. It’s also about people. Equip your marketing and analytics teams with the knowledge to understand and interpret AI-generated insights, fostering collaboration between AI systems and human expertise.
5. Iterate and optimize
AI thrives on continuous learning. Regularly review performance metrics and refine your AI-driven campaigns. Incorporate feedback loops to adapt quickly to changing market trends and consumer behaviors.
Frequently Asked Question
What is AI analytics?
AI analytics refers to the application of artificial intelligence technologies, including machine learning algorithms and natural language processing, to analyze and interpret complex data sets. This approach enables the automated extraction of insights, predictions, and patterns from data, significantly enhancing the speed and accuracy of data analysis compared to traditional methods. AI data analytics is used across various industries to optimize operations, improve decision-making, and offer personalized customer experiences by leveraging the deep analytical capabilities of AI.
How is AI used in marketing analytics?
AI is leveraged in marketing analytics to enhance customer understanding, personalize marketing efforts, and optimize campaign performance. Through ML algorithms, AI analyzes vast amounts of customer data to identify patterns, trends, and insights, enabling targeted marketing strategies. AI analytics solutions automate customer segmentation, predict consumer behavior, and personalize content delivery to improve engagement rates. AI-driven analytics also assess campaign effectiveness in real-time, adjusting strategies to maximize ROI. Natural language processing (NLP) tools interpret customer feedback and social media sentiment, providing deeper insights into customer preferences and perceptions. This comprehensive application of AI empowers marketers to make data-driven decisions, tailor experiences to individual customer needs, and achieve greater marketing efficiency.
Is AI going to replace data analytics?
AI is not set to replace data analytics but to augment it. AI enhances traditional data analytics through automation and sophisticated algorithms, allowing for more efficient processing, analysis, and interpretation of large data sets. AI data analytics introduces capabilities such as predictive analytics and natural language processing, which extend beyond the scope of traditional tools. However, human oversight remains crucial for contextual understanding, strategic decision-making, and ethical considerations. AI in data analytics represents an evolution, driving insights with greater speed and accuracy, but it works in tandem with human expertise to leverage the full potential of data.
How is AI changing marketing?
AI is transforming marketing by enabling hyper-personalization, automating routine workflows, and providing predictive analytics for data-driven decision-making. By leveraging AI, marketers can better understand customer behavior, optimize campaigns in real-time, and improve ROI through precise targeting and more efficient resource allocation.
How to integrate AI into digital marketing?
Integrating AI into digital marketing involves identifying key areas where automation and advanced analytics can add value, such as customer segmentation, content personalization, and campaign optimization. Start by selecting AI-powered tools tailored to your needs, ensuring they integrate seamlessly with your existing tech stack. Focus on leveraging AI for data analysis, predictive modeling, and automating repetitive tasks to enhance efficiency and drive better marketing outcomes.
What is the best AI for marketing?
Selecting the optimal AI solution for marketing depends on your organization's specific needs, objectives, and existing infrastructure. Improvado is an AI-powered marketing analytics and intelligence solution. Consider tools like Jasper AI and Surfer SEO for content generation and SEO optimization. It's crucial to assess each platform's features, scalability, and how well they align with your marketing strategy to determine the best fit for your organization.
500+ data sources under one roof to drive business growth. 👇
Gain insights by simply asking Improvado AI Agent any marketing question