Maximizing Profits Through Retail Analytics Insights
From sales performance and customer behavior to inventory management and marketing effectiveness, retail analytics provides the insights necessary to make informed, strategic decisions. This guide discusses the core of retail analytics, offering a roadmap to leverage your data for improved decision-making and competitive advantage.
What Is Retail Analytics?
Applications of Analytics in Retail
Retail analytics offers powerful insights that enable retailers to understand their market, optimize operations, and deliver superior customer experiences.
This section explores various applications of analytics in the retail sector, highlighting how data-driven strategies are transforming the industry.
- Customer Behavior Analysis: By analyzing transactional data, social media interactions, and in-store foot traffic, retailers gain a comprehensive understanding of customer preferences and buying habits. This analysis informs product development, store layout adjustments, and targeted marketing efforts, fostering a more personalized shopping experience.
- Inventory Management: Advanced predictive analytics models assess historical sales data, seasonal trends, and current market demand to forecast optimal inventory levels. This precision in inventory management reduces the risk of stockouts and overstock situations, balancing capital investment and customer satisfaction effectively.
- Price Optimization: Retail analytics utilizes price elasticity models and competitive analysis to identify the best pricing strategy for each product. Dynamic pricing techniques, influenced by real-time market conditions, help maximize profit margins without sacrificing competitive positioning.
- Supply Chain Optimization: Data insights streamline logistics, from procurement to distribution. Analyzing supplier performance, transportation costs, and warehouse efficiency uncovers opportunities to reduce lead times, lower expenses, and enhance the reliability of the supply chain.
- Sales Forecasting: Retailers apply machine learning algorithms to historical sales data and external factors like economic indicators and consumer trends to predict future sales volumes. Accurate sales forecasts guide inventory purchasing, promotional planning, and resource allocation.
- Personalized Marketing: Utilizing customer data, including past purchases and online behavior, retailers craft customized marketing messages and offers. Tailored communication increases engagement, drives sales, and builds loyalty by making each customer feel understood and valued.
Online vs. Offline Retail Analytics
The retail landscape is increasingly complex, blending online and offline experiences. Retail data analytics serve as a critical tool in navigating this hybrid model, with distinct applications and benefits for each channel.
Online Retail Analytics
Online retail analytics focuses on analyzing digital data to understand customer behavior, optimize e-commerce operations, and improve sales strategies in the digital marketplace. This analytics domain leverages data from website traffic, social media interactions, online transactions, and customer feedback platforms.
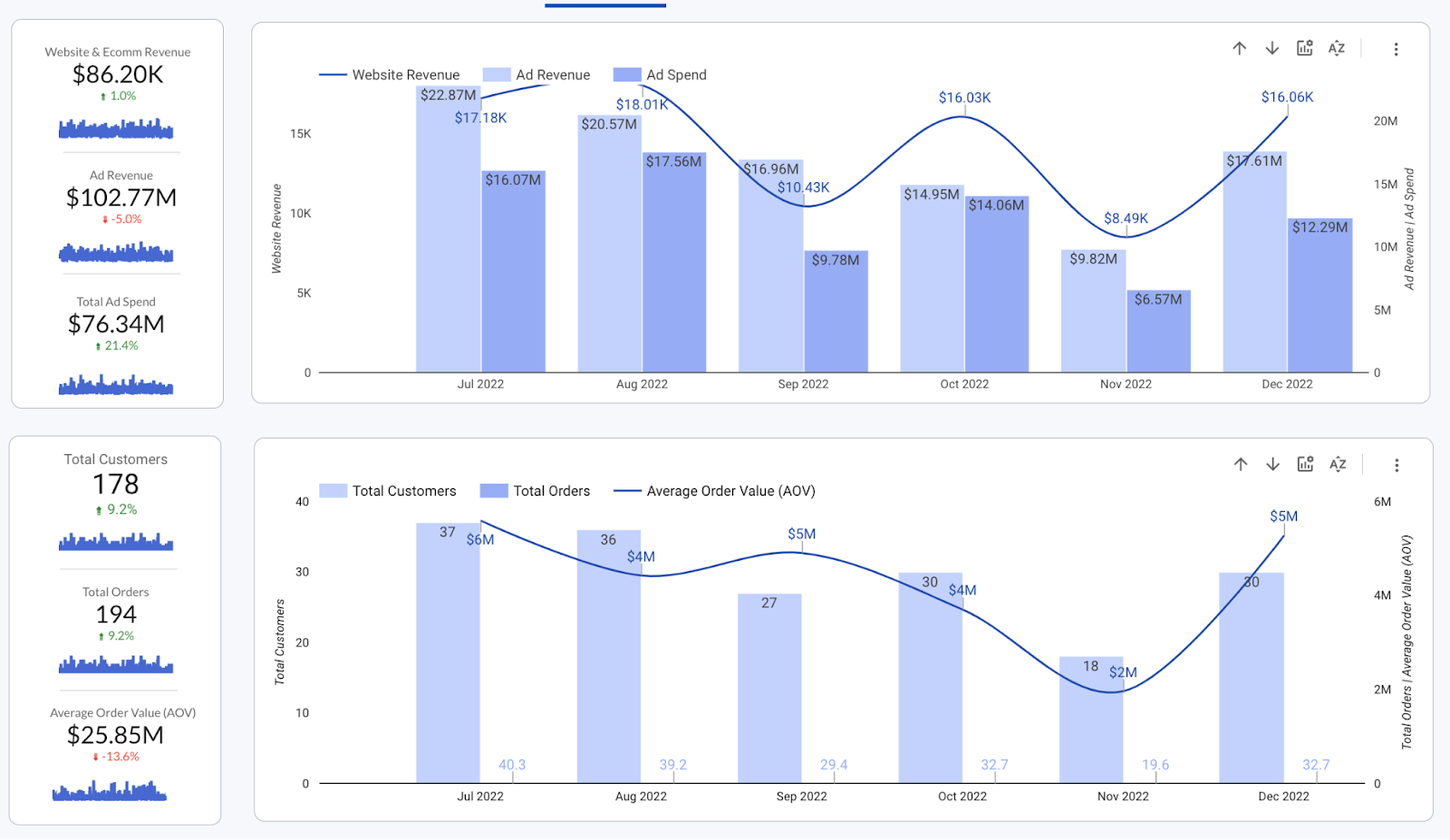
Key areas include:
- Website Traffic Analysis: Understanding how visitors navigate an e-commerce site, identifying popular pages, and pinpointing dropout points to optimize the user journey.
- Customer Segmentation: Grouping customers based on their online behavior, purchase history, and preferences to tailor marketing messages and offers.
- Conversion Rate Optimization (CRO): Analyzing user interactions to improve the online shopping experience, streamline checkout processes, and boost conversion rates.
- Social Media Analytics: Monitoring social media platforms to gauge brand sentiment, track engagement, and identify trends that can influence marketing strategies.
- Product Performance: Assessing sales data to determine top-performing products, optimize inventory levels, and make informed decisions on product listings and promotions.
Online retail analytics differentiates itself from offline analytics through several key attributes, emphasizing the unique advantages and challenges of the digital retail environment:
- Real-Time Data Access: Online analytics offers immediate insights into customer behavior and sales trends, allowing for rapid response to market changes.
- Detailed Customer Journey Tracking: Digital platforms enable the tracking of each step in the customer journey, from initial engagement to post-purchase behavior, providing a granular view of the customer experience.
- Advanced Segmentation Capabilities: The wealth of digital data supports intricate segmentation of customer bases, enabling highly personalized marketing strategies.
- A/B Testing for Optimization: Online environments facilitate real-time A/B testing, allowing retailers to quickly determine the most effective website layouts, marketing messages, and product placements.
- Social Media Insights: The integration of social media analytics into online retail strategies offers direct feedback on brand perception and the impact of social marketing efforts.
- Global Reach and Scalability: Online analytics tools are designed to manage and interpret data from a global customer base, supporting scalability and cross-market analysis.
Offline Retail Analytics
Offline retail analytics concentrates on gathering and interpreting data from physical retail environments to optimize store performance, enhance customer experiences, and drive sales.
Key areas include:
- Foot Traffic Analysis: Monitoring the number and flow of customers entering a store to gauge attraction and optimize store layout.
- Sales Pattern Analysis: Evaluating transaction data to identify best-selling products and peak sales periods.
- Customer Feedback: Collecting and analyzing feedback from in-store surveys to improve service and product offerings.
- Inventory Management: Using sales and supply chain data to optimize stock levels and reduce inventory costs.
- Employee Performance: Assessing staff efficiency and effectiveness in sales and customer service roles.
Unique attributes of offline retail analytics include:
- Physical Customer Interaction: Offers insights into customer behavior and preferences based on face-to-face interactions and observations.
- Spatial Analytics: Utilizes in-store layouts and designs to understand customer movement patterns and product placement effectiveness.
- Immediate Feedback Loop: Enables direct and immediate adjustments in store based on customer interactions and feedback.
- Tactile Product Experience: Analyzes how physical handling and viewing of products influence purchasing decisions.
- Localized Market Insights: Provides detailed insights into local customer demographics and preferences, tailoring store offerings to the community.
Core Components of Analytics in Retail
Retail analytics is not just about collecting data, it's about turning that data into a strategic asset. This section breaks down the core components that form the backbone of effective retail analytics, each playing a crucial role in transforming raw data into actionable insights.
Data Collection and Management
It all starts with data, data collection and management. Effective data management practices ensure the integrity, accessibility, and security of data gathered from various sources.
This involves:
- Point of Sale (POS) Systems: Collecting transactional data at the time of purchase, including items purchased, quantities, prices, and customer information when available.
- Customer Relationship Management (CRM) Systems: Gathering detailed customer information from various interactions, whether online, in-store, or through customer service channels, to build comprehensive customer profiles.
- Supply Chain Data: Monitoring and recording inventory levels, supplier performance, shipping details, and warehousing data to ensure efficient supply chain operations.
- Online and Social Media Analytics: Tracking online customer interactions, website traffic patterns, social media engagements, and e-commerce behaviors to gain insights into digital customer preferences and behaviors.
- In-store Sensors and IoT Devices: Utilizing technology such as RFID tags, Wi-Fi tracking, and smart shelves to collect data on customer foot traffic, product placement effectiveness, and inventory accuracy in physical stores.
Managing this wealth of data requires a structured approach, utilizing data warehouses and data lakes to centralize information, data cleaning and normalization processes to ensure data quality, and advanced analytics platforms to transform raw data into actionable insights.
Retail Business Intelligence (BI)
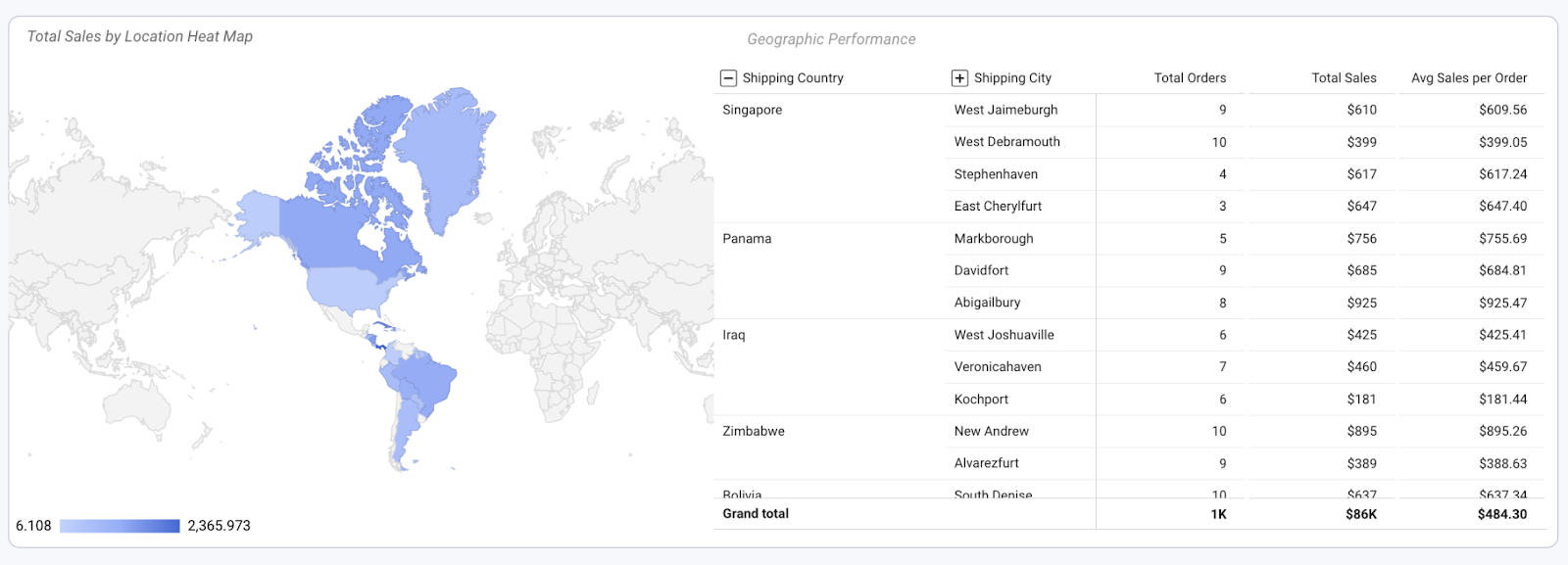
Retail business intelligence (BI) is a crucial component of analytics in retail, equipping decision-makers with data-driven insights to optimize operations, enhance customer satisfaction, and drive sales.
Essential aspects include:
- Advanced Reporting: Generates detailed reports on sales performance, customer engagement, and operational efficiency, facilitating a deeper understanding of business dynamics.
- Trend Analysis: Employs statistical methods to identify patterns within historical data, aiding in strategic planning and forecasting.
- Market Basket Analysis: Examines purchase correlations and customer buying habits to enhance product placement and cross-selling strategies.
- Loss Prevention Analytics: Analyzes transactional data to detect potential fraud and inventory shrinkage, safeguarding profits.
- Performance Benchmarking: Measures business metrics against industry standards to gauge competitive standing and identify areas for enhancement.
Retail Metrics and Key Performance Indicators (KPIs)
These metrics enable retailers to assess operational efficiency, customer satisfaction, and financial health:
- Sales Growth: Measures the rate at which sales revenue increases over a specific period, reflecting the business's growth trajectory.
- Conversion Rate: Calculates the percentage of store visitors or website users who make a purchase, indicating the effectiveness of sales strategies.
- Average Transaction Value (ATV): Tracks the average amount spent per transaction, helping to gauge pricing strategies and customer purchasing behavior.
- Inventory Turnover: Assesses how often inventory is sold and replaced over a period, highlighting supply chain efficiency and product demand.
- Customer Retention Rate: Indicates the percentage of customers who return to make additional purchases, measuring customer loyalty and satisfaction.
- Gross Margin Return on Investment (GMROI): Evaluates the financial return on inventory investments, linking inventory management to profitability.
Navigating Challenges in Retail Analytics
Implementing retail analytics is not without its challenges. From data collection and integration to making sense of vast amounts of information, retail businesses face several challenges. Understanding these obstacles is the first step toward overcoming them.
Data Quality and Consistency
One of the core challenges in retail analytics is maintaining high data quality and consistency. Data scattered across multiple sources often lacks standardization, leading to discrepancies that obstruct actionable insights.
Solution: Invest in robust data management platforms like Improvado that integrate and cleanse data from various touchpoints, consolidating data from multiple sources into a single, coherent framework. This approach allows retail marketers and analysts to rely on their data, making it possible to derive accurate insights that can enhance customer engagement and increase sales.
Integration of Diverse Data Sources
One of the primary challenges in retail analytics is the integration of diverse data sources. Retailers today collect a vast array of data from online transactions, in-store sales, customer feedback, and social media interactions. The disparate nature of these data sources can make it difficult to create a unified view of customer behavior and business performance.
Solution: Similar to the challenge of maintaining high-quality data, The solution to this challenge lies in adopting a robust data integration platform that can consolidate data from these varied sources into a cohesive, analyzable format. Such a platform should offer compatibility with multiple data formats and the ability to cleanse and harmonize data, ensuring accuracy and consistency. By effectively integrating data, retail marketers and analysts can gain comprehensive insights, enabling strategic decision-making that drives sales and improves customer satisfaction.
Improvado offers two data transformation engines:
- Marketing Common Data Model (MCDM), an out-of-the-box solution that automatically merges data from multiple channels and comes with pre-built dashboard templates for Looker and Tableau. The tool takes about two weeks to master.
- DataPrep, an enterprise-grade self-service transformation solution where you apply data rules to run transformations. It provides over 300 features and functionalities to automate lengthy analytics timelines and facilitate data discovery. The DataPrep learning curve is about two months, which is still faster and easier to navigate than digging through data with SQL.
Privacy and Security
Maintaining privacy and security poses a significant challenge. As retailers collect and analyze vast amounts of customer data, they must navigate stringent regulations and rising consumer expectations for data protection. The risk of data breaches not only threatens customer trust but also exposes businesses to legal and financial repercussions.
Solution: Implement rigorous data security measures and privacy protocols. This includes encrypting sensitive information, regularly updating security systems to defend against new threats, and ensuring compliance with data protection laws. Retailers can also adopt privacy-by-design principles, integrating data protection into the development of business processes and analytics platforms. By prioritizing customer privacy and data security, retailers can safeguard their operations while building trust and loyalty among their clientele.
Turning Data into Actionable Insights
Retailers often struggle to filter through this vast data to identify trends, patterns, and opportunities that can inform strategic decisions. The risk is that valuable insights remain buried under unanalyzed data, leading to missed opportunities for improvement and growth.
Solution: Leverage advanced analytics tools and technologies capable of processing large datasets to extract meaningful information. Implementing machine learning algorithms and data visualization techniques can help uncover hidden patterns and insights. Additionally, adopting a structured approach to data analysis, where key performance indicators (KPIs) are clearly defined, enables retailers to focus on metrics that directly impact business outcomes.
Skill Gaps
The industry's rapid adoption of technologies like AI and machine learning outpaces the available talent pool, creating a bottleneck in leveraging data for strategic advantage. This gap impacts retailers' ability to efficiently mine insights from consumer data, hindering decision-making and strategy optimization. Addressing this requires focused upskilling, recruitment of specialized talent, and possibly partnering with analytics experts to fill the immediate need.
Solution: Bridge these skill gaps by investing in continuous training and professional development for existing staff, focusing on emerging trends and technologies in retail analytics.
In Retail Analytics, Data Is Your Foundation
Data serves as the fundamental bedrock upon which all analysis, insights, and strategic decisions are built. The quality, granularity, and comprehensiveness of data directly influence the accuracy of customer behavior predictions, inventory management efficiency, and campaign effectiveness.
With Improvado, your team can make a transformative shift from disparate to analysis-ready data, while the platform's analysis and insight discovery capabilities will help you save up to 82% time, support data-driven decision-making, and drive better business outcomes.
500+ data sources under one roof to drive business growth. 👇
Leverage Improvado to turn data into insights